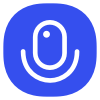
Sign up to save your podcasts
Or
In this episode of Learning Machines 101 (www.learningmachines101.com) we discuss how to solve constraint satisfaction inference problems where knowledge is represented as a large unordered collection of complicated probabilistic constraints among a collection of variables. The goal of the inference process is to infer the most probable values of the unobservable variables given the observable variables. Specifically, Monte Carlo Markov Chain ( MCMC ) methods are discussed.
4.4
9393 ratings
In this episode of Learning Machines 101 (www.learningmachines101.com) we discuss how to solve constraint satisfaction inference problems where knowledge is represented as a large unordered collection of complicated probabilistic constraints among a collection of variables. The goal of the inference process is to infer the most probable values of the unobservable variables given the observable variables. Specifically, Monte Carlo Markov Chain ( MCMC ) methods are discussed.