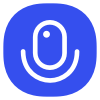
Sign up to save your podcasts
Or
Fred Sala - Efficiently Constructing Datasets for Diverse Datatypes
Building large datasets for data-hungry models is a key challenge in modern machine learning. Weak supervision frameworks have become a popular way to bypass this bottleneck. These approaches synthesize multiple noisy but cheaply-acquired estimates of labels into a set of high-quality pseudolabels for downstream training. In this talk, I introduce a technique that fuses weak supervision with structured prediction, enabling WS techniques to be applied to extremely diverse types of data. This approach allows for labels that can be continuous, manifold-valued (including, for example, points in hyperbolic space), rankings, sequences, graphs, and more. I will discuss theoretical guarantees for this universal weak supervision technique, connecting the consistency of weak supervision estimators to low-distortion embeddings of metric spaces. I will show experimental results in a variety of problems, including learning to rank, geodesic regression, and semantic dependency parsing. Finally I will present and discuss future opportunities for automated dataset construction.
5
77 ratings
Fred Sala - Efficiently Constructing Datasets for Diverse Datatypes
Building large datasets for data-hungry models is a key challenge in modern machine learning. Weak supervision frameworks have become a popular way to bypass this bottleneck. These approaches synthesize multiple noisy but cheaply-acquired estimates of labels into a set of high-quality pseudolabels for downstream training. In this talk, I introduce a technique that fuses weak supervision with structured prediction, enabling WS techniques to be applied to extremely diverse types of data. This approach allows for labels that can be continuous, manifold-valued (including, for example, points in hyperbolic space), rankings, sequences, graphs, and more. I will discuss theoretical guarantees for this universal weak supervision technique, connecting the consistency of weak supervision estimators to low-distortion embeddings of metric spaces. I will show experimental results in a variety of problems, including learning to rank, geodesic regression, and semantic dependency parsing. Finally I will present and discuss future opportunities for automated dataset construction.