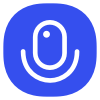
Sign up to save your podcasts
Or
Virginia Smith - On Heterogeneity in Federated Settings
A defining characteristic of federated learning is the presence of heterogeneity, i.e., that data and compute may differ significantly across the network. In this talk I show that the challenge of heterogeneity pervades the machine learning process in federated settings, affecting issues such as optimization, modeling, and fairness. In terms of optimization, I discuss FedProx, a distributed optimization method that offers robustness to systems and statistical heterogeneity. I then explore the role that heterogeneity plays in delivering models that are accurate and fair to all users/devices in the network. Our work here extends classical ideas in multi-task learning and alpha-fairness to large-scale heterogeneous networks, enabling flexible, accurate, and fair federated learning.
5
77 ratings
Virginia Smith - On Heterogeneity in Federated Settings
A defining characteristic of federated learning is the presence of heterogeneity, i.e., that data and compute may differ significantly across the network. In this talk I show that the challenge of heterogeneity pervades the machine learning process in federated settings, affecting issues such as optimization, modeling, and fairness. In terms of optimization, I discuss FedProx, a distributed optimization method that offers robustness to systems and statistical heterogeneity. I then explore the role that heterogeneity plays in delivering models that are accurate and fair to all users/devices in the network. Our work here extends classical ideas in multi-task learning and alpha-fairness to large-scale heterogeneous networks, enabling flexible, accurate, and fair federated learning.