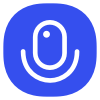
Sign up to save your podcasts
Or
Matthias Poloczek - Scalable Bayesian Optimization for Industrial Applications
Bayesian optimization has become a powerful method for the sample-efficient optimization of expensive black-box functions. These functions do not have a closed-form and are evaluated for example by running a complex economic simulation, by an experiment in the lab or in a market, or by a CFD simulation. Use cases arise in machine learning, e.g., when tuning the configuration of an ML model or when optimizing a reinforcement learning policy. Examples in engineering include the design of aerodynamic structures or materials discovery.
In this talk I will introduce the key ideas of Bayesian optimization and discuss how they can be applied to tuning ML models. Moreover, I will share some experiences with developing a Bayesian optimization service in industry.
5
77 ratings
Matthias Poloczek - Scalable Bayesian Optimization for Industrial Applications
Bayesian optimization has become a powerful method for the sample-efficient optimization of expensive black-box functions. These functions do not have a closed-form and are evaluated for example by running a complex economic simulation, by an experiment in the lab or in a market, or by a CFD simulation. Use cases arise in machine learning, e.g., when tuning the configuration of an ML model or when optimizing a reinforcement learning policy. Examples in engineering include the design of aerodynamic structures or materials discovery.
In this talk I will introduce the key ideas of Bayesian optimization and discuss how they can be applied to tuning ML models. Moreover, I will share some experiences with developing a Bayesian optimization service in industry.