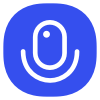
Sign up to save your podcasts
Or
This episode of Recsperts features Justin Basilico who is director of research and engineering at Netflix. Justin leads the team that is in charge of creating a personalized homepage. We learn more about the evolution of the Netflix recommender system from rating prediction to using deep learning, contextual multi-armed bandits and reinforcement learning to perform personalized page construction. Deep content understanding drives the creation of useful groupings of videos to be shown in a personalized homepage.
Justin and I discuss the misalignment of metrics as just one out of many elements that is making personalization still “super hard”. We hear more about the journey of deep learning for recommender systems where real usefulness comes from taking advantage of the variety of data besides pure user-item interactions, i.e. histories, content, and context. We also briefly touch on RecSysOps for detecting, predicting, diagnosing and resolving issues in a large-scale recommender systems and how it helps to alleviate item cold-start.
In the end of this episode, we talk about the company culture at Netflix. Key elements are freedom and responsibility as well as providing context instead of exerting control. We hear that being really comfortable with feedback is important for high-performance people and teams.
Enjoy this enriching episode of RECSPERTS - Recommender Systems Experts.
Chapters:
Papers:
General Links:
5
33 ratings
This episode of Recsperts features Justin Basilico who is director of research and engineering at Netflix. Justin leads the team that is in charge of creating a personalized homepage. We learn more about the evolution of the Netflix recommender system from rating prediction to using deep learning, contextual multi-armed bandits and reinforcement learning to perform personalized page construction. Deep content understanding drives the creation of useful groupings of videos to be shown in a personalized homepage.
Justin and I discuss the misalignment of metrics as just one out of many elements that is making personalization still “super hard”. We hear more about the journey of deep learning for recommender systems where real usefulness comes from taking advantage of the variety of data besides pure user-item interactions, i.e. histories, content, and context. We also briefly touch on RecSysOps for detecting, predicting, diagnosing and resolving issues in a large-scale recommender systems and how it helps to alleviate item cold-start.
In the end of this episode, we talk about the company culture at Netflix. Key elements are freedom and responsibility as well as providing context instead of exerting control. We hear that being really comfortable with feedback is important for high-performance people and teams.
Enjoy this enriching episode of RECSPERTS - Recommender Systems Experts.
Chapters:
Papers:
General Links: