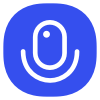
Sign up to save your podcasts
Or
Welcome our guests to the podcast. We are going to discuss Asset Answers in today's episode. However, briefly about our guests:
Yanpei has a background in telecom and smart grid. Currently is working as a product leader at Asset Answers.
Manjish is the service lead for Asset Answers and has been in the maintenance and reliability for 15 years building CMMS and analytic software on top of the CMMS)
In this episode we covered:
Many organizations have data but not information. Have you witnessed such?
In many industries. They do not have information on how a failure was detected, what kind of failure it was and, was it a people, system, or process related failure?
What are the main causes of suspect data?
The causes include:
The sites might have different functional location hierarchies I.e. areas, sites, systems, units such that: what might be a unit is considered a system in another.
Does the data have to be perfect to start?
No it doesn't. The solution we provide is to focus on the purpose of the data. You can calculate key performance metrics using the small available data.
How do organizations know the specific data points needed?
First, understand the organization's goals– perhaps to optimize resources or reduce equipment failures. Determine:
Can we still gain insight into an asset using things like the Law of Big Numbers?
You can work backwards once you have decided and defined the purpose of the data. You do not need absolute numbers. For instance, measuring the value of allocating resources on working on one equipment versus another.
How do organizations select the right asset to work on once they have good data?
There are multiple ways of selecting a bad actor. For instance:
At Asset Answers, we have peer benchmarking analytics to compliment the internal view of key performance metrics. It provides an external view of the businesses in the same industry producing the same product.
How does peer benchmarking analytics work?
Asset Answers has been built by:
It is basically the aggregation of data to analyze it at different levels (site, area, equipment class etc). The biggest challenge is standardization. It is an easy way to find bad actors.
What action drive improvements on identified bad actors?
Asset Answers tells you where to focus on. Actions depend on the asset strategy in place. We provide another tool called PM Diagnostics where both instances of reactive and proactive are counted.
The two are sketched on an axis (proactive maintenance on X-axis and reactive on Y-axis) to create 3 categories:
Can we calculate ROI for all these using Asset Answers?
We installed an equipment tracker that stores data on historical (before) and after improvement activities to show ROI.
Does it consider downtime avoidance?
Depends on the data being tracked in the CMMS and its quality. Can be used to measure maintenance versus downtime cost.
Key takeaway
Eruditio Links:
Manjish and Yanpei Links:
Download RSS iTunesStitcher
Rooted In Reliability podcast is a proud member of Reliability.fm network. We encourage you to please rate and review this podcast on iTunes and Stitcher. It ensures the podcast stays relevant and is easy to find by like-minded professionals. It is only with your ratings and reviews that the Rooted In Reliability podcast can continue to grow. Thank you for providing the small but critical support for the Rooted In Reliability podcast!
The post 276 – Driving Asset Answers with Suspect Data with Manjish and Yanpei appeared first on Accendo Reliability.
4.8
2828 ratings
Welcome our guests to the podcast. We are going to discuss Asset Answers in today's episode. However, briefly about our guests:
Yanpei has a background in telecom and smart grid. Currently is working as a product leader at Asset Answers.
Manjish is the service lead for Asset Answers and has been in the maintenance and reliability for 15 years building CMMS and analytic software on top of the CMMS)
In this episode we covered:
Many organizations have data but not information. Have you witnessed such?
In many industries. They do not have information on how a failure was detected, what kind of failure it was and, was it a people, system, or process related failure?
What are the main causes of suspect data?
The causes include:
The sites might have different functional location hierarchies I.e. areas, sites, systems, units such that: what might be a unit is considered a system in another.
Does the data have to be perfect to start?
No it doesn't. The solution we provide is to focus on the purpose of the data. You can calculate key performance metrics using the small available data.
How do organizations know the specific data points needed?
First, understand the organization's goals– perhaps to optimize resources or reduce equipment failures. Determine:
Can we still gain insight into an asset using things like the Law of Big Numbers?
You can work backwards once you have decided and defined the purpose of the data. You do not need absolute numbers. For instance, measuring the value of allocating resources on working on one equipment versus another.
How do organizations select the right asset to work on once they have good data?
There are multiple ways of selecting a bad actor. For instance:
At Asset Answers, we have peer benchmarking analytics to compliment the internal view of key performance metrics. It provides an external view of the businesses in the same industry producing the same product.
How does peer benchmarking analytics work?
Asset Answers has been built by:
It is basically the aggregation of data to analyze it at different levels (site, area, equipment class etc). The biggest challenge is standardization. It is an easy way to find bad actors.
What action drive improvements on identified bad actors?
Asset Answers tells you where to focus on. Actions depend on the asset strategy in place. We provide another tool called PM Diagnostics where both instances of reactive and proactive are counted.
The two are sketched on an axis (proactive maintenance on X-axis and reactive on Y-axis) to create 3 categories:
Can we calculate ROI for all these using Asset Answers?
We installed an equipment tracker that stores data on historical (before) and after improvement activities to show ROI.
Does it consider downtime avoidance?
Depends on the data being tracked in the CMMS and its quality. Can be used to measure maintenance versus downtime cost.
Key takeaway
Eruditio Links:
Manjish and Yanpei Links:
Download RSS iTunesStitcher
Rooted In Reliability podcast is a proud member of Reliability.fm network. We encourage you to please rate and review this podcast on iTunes and Stitcher. It ensures the podcast stays relevant and is easy to find by like-minded professionals. It is only with your ratings and reviews that the Rooted In Reliability podcast can continue to grow. Thank you for providing the small but critical support for the Rooted In Reliability podcast!
The post 276 – Driving Asset Answers with Suspect Data with Manjish and Yanpei appeared first on Accendo Reliability.