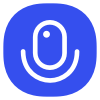
Sign up to save your podcasts
Or
Andrew Amann, CEO and Co-founder of NineTwoThree Studio, is driven by a passion for building great products and embracing the entrepreneurial journey alongside his team and clients.
We learn about Andrew’s journey as a founder and his philosophy of finding joy in the process. He introduces The AI Operating System, a practical framework that helps companies adopt AI with clarity and speed. The system begins by building an internal knowledge base, then moves into deploying simple AI agents to automate repetitive tasks, and culminates in managing these agents through a Super-Agent that orchestrates workflows and interacts with external systems using natural language.
Good day, dear listeners, Steve Preda here with the Management Blueprint Podcast. And my guest today is Andrew Amann, the CEO and co-founder of NineTwoThree Studio, an AI consulting agency, building products for funded startups and established brands such as Consumer Reports, NPR, and Experian. Andrew, welcome to the show.
Thanks for having me, Steve. It’s a pleasure to be here.
Great to have you. And I will start with my favorite question as always, what is your personal “Why,” Andrew, and how are you manifesting it in your business?
Sure, so we’ve been at this for 12 years, and I believe that many entrepreneurs would answer that question depending on the year that they’re in. As you grow, my personal”Why” originally was to build the biggest company to be on the tallest building in Boston and to have the logo basically replace Fenway Park. And that dream has has sailed. I don’t think I’m interested in that anymore. It seems aspirational and I think necessary when you’re starting a company to have those levels of aspirations and dreams because you have to run through things that most people wouldn’t and you have to achieve things that most people would give up on.
So the journey of entrepreneurship, I’ve discovered that my “Why” is to be part of the journey. I’ve read a lot of books recently, basically around the philosophy of the process of like Siddhartha is a great example, or The Alchemist, or even Life of Pi. That’s been my last year of inner research about what I want to be when I grow up. And what I discovered in all three of those books is the journey is what matters, the story is what matters, and the process of success, the process part is what matters. And many entrepreneurial friends, they sell their business and they lose that aspect of their lives because they forget that the process was what was fun.
The ability to learn, the ability to grow, and the ability to be part of the team is what I truly admire. So when I was asked this question in a CEO group, they said, Andrew, what do you want to do next? I said, I kind of want to do the same thing I’m doing now. And they said, well, who do you wanna work with? And I said, the teammates that I currently have. And they said, well, what type of clients do you want? And I said, the clients we currently have. And so I think my “Why” is just kind of living in the moment, being part of the process, being successful, but also supporting the teammates that we have so that they can be prosperous, that they can find their purpose. They can be great problem solvers, but they can also be great providers for their family. And so my “Why” is to support that journey and that process that we’re going through right now.
Wow. Okay. Well, you look very young for having such a wise “Why,” but it’s good. You shortcut the process. I do agree that the journey is the destination, perhaps. It definitely is very important. And as an investment banker, I can testify that sometimes you saw businesses for people and then they fall into depression because they lost their identity. They didn’t know how to recreate the success that they were having along the way. And so that’s pretty awesome. So having said that, you are in a very highly evolving area, which is AI. You’ve worked very actively in AI. I mean, you do other stuff as well. But we talked about AI on our pre-call and I was very intrigued by the framework that you’ve developed around what you call the AI Operating System. So what is an AI Operating System and how do you get one in your company?
Sure. So I come from a supply chain background. I built nuclear submarines for the first five years of my life. And when you build for the government, you have to learn processes, but you also have to learn throughput. One of my favorite books of all time is The Goal by Eliyahu Goldratt. And it’s just a phenomenal book that teaches you about throughout and bottlenecks and relieving those bottlenecks to get to the next step so that you can ultimately achieve the profit. So with AI, we’ve been at this for eight years now. We’ve been developing a process and a strategy of how to implement AI into businesses.
What we’ve discovered is that CEOs are very scared of deploying something new, something that they don’t know and something that their friends or CEO friends are not doing the same way that they are. It was very easy to say, let’s move to the cloud because it had such a promising effect on the company that they could work from anywhere. The cloud would store all the data, they wouldn’t have to worry about servers going down. And all of their friends are moving to the clouds, all the other CEOs are moving to the cloud. So it was a transition that I think a lot of organizations took upon, whether it was Microsoft or Amazon, it didn’t matter. The movement to the cloud mattered. From our standpoint, AI is not so simple. AI becomes a very individualistic goal. It is something that your IP or your company has this fingerprint around, and you’re trying to AI that, which means it’s something different from your friends. It’s different from other companies. It’s a different organization.
And because everybody’s fingerprint is different, everybody’s adoption of AI is going to be different. But we’ve created a process of a workflow that you go through, we call it, you start with exploration, which is free, where you come through us and you try to figure out exactly what you’re trying to solve for. We then go through what we call product strategy and engineering discovery. And what that specifically does is diagnose what the problem is inside of the company and what the return on investment would be if we solve that problem. Now, I’ve been kind of making fun of Deloitte and Accenture for the last year because those two companies have this strategy session as well. It costs a million dollars, and they have great success with it. I’m not touting them for their success because a lot of companies see the value in hiring those types of consultants. What I am kind of knocking on is that to find value with AI should not take more than one or two months. The return on investments that we’re seeing are in the seven figures or even eight figures with a $200,000 investment. And AI changes the game for that initial discovery phase for what I call the strategy sessions. Because within a month, we should not only be able to determine what the ROI is, we should be very accurate with that. Because when AI is deployed properly, it either decreases costs or increased revenues. And we’ve seen that two dozen times in a row at this point. And we have the track record to prove that.
But the process should point out that once you solve for the KPIs, or once you’re trying to determine what the ROI is of this investment, it is now a system that you put in place to develop or implement that solution. It is no longer a what if, let’s test these three avenues. AI allows you to prototype, experiment, and deploy much quicker to get those results. So we like to say that every project we’ve worked on in the last, I would say, six to seven months has been less than four months of effort to get to a production release. We don’t build MVPs anymore, we don’t build prototypes, we build first production releases that get people into the system, working with it, testing it, and most importantly, seeing what the return on investment truly is based on the estimate. And if we achieve that return on investment, well, there’s more money to be had to keep building AI solutions. So everybody wins.
Okay, so let’s unpack this. So what does it mean to find the ROI? Give me an example of a project that you had kind of a hypothesis or an initial idea, and then you tested it and it showed an ROI and then you scaled it up. What does it look like?
Sure. So we had a consulting firm that worked for legal, it was a legal consulting firm. They help lawyers and paralegals determine what documentation and what information went into whether it’s prison reports or people that were sentenced to prison. We were able to double the revenues of that company and reduce the costs by about 90%. Now, when that happens, you’re basically allowing individuals to work the same way they did before. Nobody was fired. Everybody’s still supporting the initial goal, but they’re creating more revenue with the efforts they’re putting in. They’re also doubling down on what they’re good at, and they’re removing their waste. So, when an AI solution comes in, it allows all the consultants on the team, or a lot of the people that are doing the research to start getting this energy around what a new process looks like when built upon AI. And so this specific company, I mean, they have seen great success from not just the product itself, but from the time it takes to build the report.
Okay. So can you be more specific? What is it that they do differently with these reports? And how did you cut the cost by 90% if you kept everyone on the payroll? How is that even possible? What kind of costs could you eliminate?
Yeah, so the process that works very well with AI is when you’re looking at research or you’re looking at information of how a person is just looking at thesis paper or college kid looking through a thesis paper, it takes a lot of time to gather all of the links and the resources needed to write that thesis paper. And so let’s say it takes 10 hours to gather the information, another 10 hours or five hours to start writing that outline, then another few hours to take that outline and turn it into segments. And then finally, the writing of the thesis paper is your accumulation of all of that knowledge into one final paper. All of those steps can now be almost replaced by AI, except for the final writing piece. That piece in which the individual needs to take the knowledge that they’ve retrieved and make something new out of it.
That is where AI does not have a great success factor. It hallucinates. It doesn’t know what the human is trying to draw out of the research. And that’s where humans are so good. We can connect dots that don’t seemingly need to be connected, and we can make that connection. I mean, Malcolm Gladwell is phenomenal at connecting two unknown dots and then making you believe that that’s the truth. I’m not saying that he’s falsifying it, but he’s doing a great job at analogies and summarizing how things relate to each other. And humans are really good at that. But the cost of research, the cost of outlining, the cost of brief, that goes to the AI. So you’re reducing the cost of needing to do that aspect of it. All of the employees are still doing the human aspect of it, which is reporting the information necessary back to the client or the legal client and saying, this is what we found.
This is what the research told us. And instead of taking 20, 30, 40 hours to do that, you can do that in one hour. And so, you’re creating revenue, and the cost of performing those reports just goes from 20 hours or 30 hours down to one. More importantly, you can do 10, 20, 30, 40 reports where you used to be able to do two a week. And so that’s how the costs go down. It’s not that it’s like fabricated costs. Those costs would have existed if you got 40 on your desk, but they don’t exist because you have time to take on 40 new reports that you never did before.
Yeah. So, so essentially the cost per project goes down by 90%.
That’s right.
The actual cost of the company doesn’t, it stays the same perhaps.
That’s right.
And the revenue increases dramatically.
That’s right. I mean, cost is always a determination of it’s a ratio. COGS or operation expense, it’s always a ratio of revenue. So as you increase your revenue, as a team draws in more, you’re moving the bottleneck from the operations to the very end, which is the sales. And so, if sales is the limiting factor of how many reports you can do, well, no longer is that the case because the number of reports you can do, the throughput is much higher. So you can increase sales and have the same amount of throughput or increased throughput.
Yeah, okay, that makes sense. So that’s a really great way as a certain processes, AI can essentially streamline it, save a lot of time and let people do the stuff that only people can do for now, which is synthesizing, perhaps, certain concepts. So what are the types of AI solutions that you can deploy? So this is definitely creating research is one type. In our pre-call, we talked about AI agents, we talked about orchestration. So what kind of approaches there are big picture for AI deployment that someone could consider.
Yeah, there’s really three areas and they build on each other of where AI is going. The third one is not talked about much and we’ll get there in a second. But the first one is every company has a cadence, an IP, a knowledge base, a place in which they spend most of their time, whether it’s on Google Drive, sharing documents, trading information back and forth, communicating in Slack, all of that creates what we call culture, company culture. And Microsoft’s culture is very different than HubSpot’s culture. In fact, HubSpot has a glossary of three letter abbreviations that I don’t even know what 90% of them mean. But if you work at HubSpot, you surely do. And there are many cadences and there’s many cultures that have differences between their competitors. And what’s important about that is that it’s documentable, it’s proceduralized, and it’s also very, if you get hired into one and you move to another, you might not fit, even though you’re in the same industry. And what I’m trying to point out is that that culture and that cadence is an IP.
And every company has one, and they’ve been drilling for years and years and years, what they’re very specifically good at. They’re good at customer service. They’re maybe the cheapest price out there. Maybe they’re very good at how they relate to their clients. Maybe they go on site and they do certain consulting things. Maybe it’s a SAS product or maybe it’s a dropshipping and they’re the best dropshippers because they have two day delivery. Each one of those matters and that is why a knowledge base is the first place that AI should be built for every single one of these companies. When Amazon heard about large language models, guess what they did?
They built an internal large language model that only Amazon employees can use so that when you’re writing an email, you can say, what are the 900 emails that were similar to the one I’m trying to write internally for Amazon? Not around the world, not competitors, not other SaaS products. Internally on Amazon, what were the 900 emails related to how I wanted to ask for a new hire to get a promotion? That’s a very Amazon way of asking. They have a different promotion plan than most companies do. So to train that knowledge base on your internal IP and your company is the first step that every company should be doing. Now, Co-Pilot and Google Workspace are trying to enter into this space, and I would still argue that if you’re really interested in building AI, you need a consulting firm to come in and help you organize this the right way, because otherwise you’re just going to be what Google wants or what Microsoft wants. But that is 100% the first step. Once you have that knowledge base secured, you now can go to the second aspect, which is the agents.
You can deploy agents to do things like chat. You can deploy them to do invoice reconciliation. Or you can do, which our favorite is, workflow automation. When somebody passes documents from one person to the next, there’s an opportunity to reduce that workflow and either approve it or validate it or disapprove it or not allow it, whatever word you want to use to reduce the back and forth paperwork that humans have inside of companies. So agents can be deployed, they can have specific tasks, they can perform different functions, and that becomes your second introduction to AI. And it doesn’t work unless you have a knowledge base to relate back to. Like if you just deployed an agent, it’s not going to know how to act or how to perform or when to stop or when to misbehave or when to not behave or behave. So that agent thing becomes your second wave. Now the third one is where we’re heading. And that is when companies communicate to other companies through a large language model or through what we’re calling natural language.
No longer are APIs going to be the plumbing of the internet. Natural language is going to communicate between two pieces of software. So not just engineers can ask a question, but anybody in the organization can ask a question about their software. What is the invoice related to? How many orders are coming on plane 72 so I can get the shipments out the door? What is my cadence for understanding the exact processes of what I’m trying to do right now? What are the steps needed for me to do process A, B, and C? That is when I have to communicate with software, other systems, or even competitors. And as a product manager, designer, finance, HR, or developer, natural language or English should be the code of this plumbing line. And that’s what everybody’s building. That’s where we’re heading. That’s when a phone communicates to a laptop. That’s when your wife texts you, pick your kid up at six o’clock. And she doesn’t tell you the details, but it understands your calendar. It understands that every six o’clock ninja class happens and you need to go pick your kid up.
These things are coming down the pipeline and they’re coming fast and that only happens when a knowledge base is set up for either yourself or your company. Agents are deployed, which we’re calling MCP Service, but like basically these places are secure for agents to ask questions on your behalf and thirdly, for it to go outside the walled garden of your personal data and retrieve information for you.
Okay, so I’m trying to relate this to the use of ChatGPT, and maybe that’s not a good way to do it. But I’ve got a ChatGPT teams subscription, which is supposedly private. So what I do is, ChatGPT knows about me, knows what I do, knows the kinds of clients I’m working with, and understands some of the products I have. And then I basically ask it to do certain things. So I wonder if that’s similar to deploying agents. So now it knows my knowledge base. Maybe it’s not completely built out like it should be, but at some level it knows. So I can ask questions and then even create workflows. But the third step is to communicate with software, other competitors, and so on. I don’t understand exactly how that works. So what is the step between agents and this natural language communication? What is the missing step that needs to be breached to get there?
Sure, so in relationship to ChatGPT, the first thing that you’re doing is creating a knowledge base of things you know so that ChatGPT when it prompts back to you and retrieves the answers for you, it’s retrieving in context that you understand. So while you have a mini knowledge base and you’re putting information into a project or to Microsoft Co-Pilot, that knowledge base is what’s informing ChatGPT of the answer it should be giving. That’s step one. Step two is the agent that you are, like a web agent. When it crawls the Internet, that is an agent going out to crawl the web, looking at the first eight links of Google, grabbing information back and bringing that to you to say, hey, I searched the Internet for you and I found this answer.
That’s an agent. But you can also imagine an agent with your Excel documents. Maybe you put in a number of shipments every single month into column B2. Well, an agent can do that for you after the shipment is retrieved from your invoicing documents. So because it has a knowledge base and because it has a knowledge base and because it has information about your shipping reports and your books, you can write an agent to say every time we retrieve our shipments, go up to this Excel sheet and fill in the correct number. But it’s not just an API, it’s smart enough to know that a half a shipment matters and it should be 7.5. It’s smart enough to know that if you have a negative shipment, well, that also means that the stuff is on the way, it just hasn’t been fulfilled yet.
It’s basically an automation that I create that will do repetitive tasks.
That’s right, it’s automation to create, and any agent can do, there can be small agents, they can be teeny tiny to do something simple, and they could be massive agents, like a chatbot that answers customer queries. Those are the level of agents. And the third one is going outside your walled garden. The best example that I’ve given repeatedly is you’re standing on the corner, you’re at your house and you’re ready to go to the airport. You should be able to say, I would like a ride to the airport and I would like to stop and get maybe some deodorant or maybe you want to get a sandwich on the way to the airport. And I would also like to get there a little bit early so I can have a presentation. I can do a phone call from the lounge.
Now to do that, two things need to be happening that don’t happen right now. One is security and privacy. The other one is the ability for these agents to send information out discreetly on your behalf. In this scenario, the ultimate goal is that agent goes out and crawls Uber, locates an Uber car for you and brings it to your house at the specific time needed for you to go to the airport. The second one would be to understand the mapping system between your house and the airport to figure to stop at CVS and maybe just stop at a sandwich. Most importantly, a sandwich shop that you’ve been to before that you like, that you’ve said before you’ve enjoyed. And then lastly, to understand your calendar, gather the meeting that you’re trying to be in, make sure that you’re at the airport with plenty of time for you to get through security and to your meeting. That requires your agents to communicate with other servers that you don’t have privy to or information to, or maybe you have a login to Delta and maybe you have a login to get to their security and make sure you’re validated. But these agents need to have security when they go out, just like an API call has a secret key.
Those agents are going to have to go out and on your behalf, act on what you want them to do and securely keep your information away from prying marketers that then intercept that. And all of a sudden, Fluffy’s car service shows to pick you up. And if Fluffy’s car service shows up and you were expecting Uber, you’re not going to be a happy client. But what happened there is Fluffy’s car service decided that they had a better marketing team and they were able to intercept these agents and deliver cars all around the world. And that’s what can start happening if we don’t keep these things secure when they go out and make the requests on our behalf.
So that sounds like a lot of complexity. How far are we from these super agents that can use my personal information?
So on Google, it’s already here. It’s unfortunate that iPhone has such a large footprint in America because I don’t think people are realizing the advancements Google has made in the last two months on their Gemini platform. I, right now, can book a trip, I can play a song, I can just have Spotify playing in the background, which seem relatively general, but when you have a large language model that understands your calendar, Gmail, your text messages, and also your internal drive, like my personal drive, it knows information about me while I’m requesting in my voice assistant. And so Google has made these great advancements with what they call Gems in the last month. I imagine that we’re gonna see an Uber Gem, an Airbnb Gem, all of that is going to come into this Google ecosystem. And they’re three years ahead of Apple at this point, in my opinion.
Wow, that’s pretty crazy. Now, what about the security aspect of it? Because obviously, I mean, more and more, I tried to download something, it always asked me to confirm that I trust this company, it can use all my information, it has access to my email and all that stuff. And it’s very scary. Sometimes I say, no way, I’m not going to do that. It’s just feels too intimidating to do this. And then plus people are concerned about government listening in. How can this thing can grow without people being overly exposed to their information?
Yeah, so recently, we’ve always had this problem and we haven’t been able to name it, just like we weren’t able to name what Retrieval-Augmented Generation was. There was always these things that we were doing inside of our company, as we’re AI consultants, we build these models all the time. And we were doing exactly this, we just didn’t have a name for it. And recently, Claude, I believe, was the first to coin the phrase Model Context Protocol. It’s a standardized way for AI applications, which basically like think of large language models, that to interact with external tools and data sources. And they act as like a universal connector. So think of like a USB-C and a USB-C port. You have the USB-C port and you want to plug into Uber and Airbnb and the receiving end, and you need a USB-C receiver to plug into. Well, it secures your privacy. It secures your information.
It secures who you are and kind of tokenizes you. And so, then every time you want to use an Uber request, the large language model can use that Uber request securely to retrieve your information about your Uber and back to you. So it’s kind of the new plumbing that’s happening across the internet. That MTP server is becoming the terminology that we’re using of how that all works. It’s the plumbing of APIs for large language models.
Yeah. And so all I have to do is trust Google who owns all these products?
No, I’ve been speaking about this. I hope that companies like Perplexity or Claude are, they have the ability to get onto hardware. And so, right now, Google and Apple have a massive advantage because they have the hardware and the voice assistance built into those devices. So they, for sure, have a duopoly around where most people are going to be sharing their information. And Google already has been doing it for me for a few months now. I hope that Perplexity and Claude and even OpenAI comes out with hardware that I can select that piece of hardware where I maybe own end-to-end encryption about the device in my large language model so that I can control the data security. So that little checkbox that you’re saying when you ask for your calendar to be shared, maybe that checkbox is something that you truly can protect against and Google doesn’t just know about it anyways.
Yeah. I mean, even when companies request me to sign in through Google, I always think, okay, what is it going to leak from Google to this company? I’m giving some permission. Do I really understand exactly what permission I’m giving? It’s just a very uncomfortable situation.
Exactly. And I think that hopefully can be reversed because if you have all the information necessary on your phone through the large language model, hopefully we can call apps and retrieve like if I wanted to buy a toaster, I don’t need to go to Amazon to search toaster to find one. I should be able to search my voice assistant for the best toaster, decide that it’s on Amazon and click purchase and the agent should take care of the rest from there.
Okay. Well, brave new world. Definitely very exciting. So I love this clarity around building out the AI. So first, the knowledge base. Create your own knowledge base and train your own AI, and then deploy agents. So essentially automate all your repeatable processes so that you can save all the energy to bigger tasks. And then maybe leverage something like Gemini that will coordinate multiple of these agents, super agent kind of thing. Maybe I misunderstand it, but it feels to me that it’s kind of a coordination of multiple agents, it’s kind of the manager of the agent workforce that is going to be doing things for you and you just give them instructions. It’s essentially a delegate to this personal integrator, whatever you want to call it.
Exactly. No, you got it. That’s the process.
That’s, that’s awesome. Okay. So our time is coming to the end here. Let me ask you one more question here. So as an entrepreneur, it was very interesting how your “Why” evolved. You wanted this tallest building with the logo and now you really want to journey and to enjoy working with the great people and working on exciting projects. What do you feel is the most important question any entrepreneur should be asking themselves?
I think the obvious one to me now is the vocation. Like chasing money is one thing. But if you truly like what you do, you shouldn’t have to worry about the money because you should be able to find enough work in that area to be successful. So, there’s a lot of entrepreneur advice out there of chasing dropshipping or chasing different areas that are the hot topic of the day. And it seems like a quick way to make money. But if you truly can’t see yourself being there for 10, 20 years, it might not be worth the effort to go into a market like that. And I also would say the same thing about TAM, like if your total addressable market is just not that big, and it’s a little tiny market, it might not be advantageous for you to put all your energy into that.
I would look at your next 10 years or 20 years and figure out where do you really enjoy being, who do you enjoy talking to, who do you enjoy working with, and what type of work do you wanna do in 10 years, and then start learning in that direction. Because if you work the other way and chase the money, you’ll end up in an industry with all these skills that might not be fruitful for a long period of time. So I truly love what I do. So I’m in the camp of finding an area. I love consulting. I love being an apprentice. I love building products. And being a consultant is all three of those, especially an AI consultant. I think people should look at that first and figure out, all right, what would excite me in 10, 20 years? And is there a big enough market that I can make my million dollars in that area and work backwards and try to figure out how to learn to be in that vocation?
Yeah, I think it’s very smart, especially because if you were to choose for the money, then it’s gonna be really hard for you to be successful without not loving what you do.
Exactly right.
So it might not even work. I mean, maybe it’s not just the fleetingness of those areas. Maybe dropshipping is a fleeting thing. I don’t know. It’s a hype thing, but you can’t even get there without loving the stuff.
I agree.
Yeah, that’s cool. All right. Well, so Andrew, if people would like to build their own AI Operating System? Where can they find you and how can they get more information or get started?
Sure, so I’m on LinkedIn. I post once a day. You can message me there. I read all the comments. Andrew A-M-A-N-N, and my company is NineTwoThree. You can fill out a form if you’re interested in a project, but for the most part, following us is the best thing I can ask for.
Well, definitely go and follow Andrew Amann, check his posts and also get immersed in AI because it’s moving very fast and you’re gonna miss the boat if you don’t. And if you enjoyed this episode, make sure you follow us on YouTube and give us a review on Apple Podcasts and join next week when an exciting entrepreneur is going to bring a framework to us. So, thank you for coming, Andrew, and thanks for listening.
5
3535 ratings
Andrew Amann, CEO and Co-founder of NineTwoThree Studio, is driven by a passion for building great products and embracing the entrepreneurial journey alongside his team and clients.
We learn about Andrew’s journey as a founder and his philosophy of finding joy in the process. He introduces The AI Operating System, a practical framework that helps companies adopt AI with clarity and speed. The system begins by building an internal knowledge base, then moves into deploying simple AI agents to automate repetitive tasks, and culminates in managing these agents through a Super-Agent that orchestrates workflows and interacts with external systems using natural language.
Good day, dear listeners, Steve Preda here with the Management Blueprint Podcast. And my guest today is Andrew Amann, the CEO and co-founder of NineTwoThree Studio, an AI consulting agency, building products for funded startups and established brands such as Consumer Reports, NPR, and Experian. Andrew, welcome to the show.
Thanks for having me, Steve. It’s a pleasure to be here.
Great to have you. And I will start with my favorite question as always, what is your personal “Why,” Andrew, and how are you manifesting it in your business?
Sure, so we’ve been at this for 12 years, and I believe that many entrepreneurs would answer that question depending on the year that they’re in. As you grow, my personal”Why” originally was to build the biggest company to be on the tallest building in Boston and to have the logo basically replace Fenway Park. And that dream has has sailed. I don’t think I’m interested in that anymore. It seems aspirational and I think necessary when you’re starting a company to have those levels of aspirations and dreams because you have to run through things that most people wouldn’t and you have to achieve things that most people would give up on.
So the journey of entrepreneurship, I’ve discovered that my “Why” is to be part of the journey. I’ve read a lot of books recently, basically around the philosophy of the process of like Siddhartha is a great example, or The Alchemist, or even Life of Pi. That’s been my last year of inner research about what I want to be when I grow up. And what I discovered in all three of those books is the journey is what matters, the story is what matters, and the process of success, the process part is what matters. And many entrepreneurial friends, they sell their business and they lose that aspect of their lives because they forget that the process was what was fun.
The ability to learn, the ability to grow, and the ability to be part of the team is what I truly admire. So when I was asked this question in a CEO group, they said, Andrew, what do you want to do next? I said, I kind of want to do the same thing I’m doing now. And they said, well, who do you wanna work with? And I said, the teammates that I currently have. And they said, well, what type of clients do you want? And I said, the clients we currently have. And so I think my “Why” is just kind of living in the moment, being part of the process, being successful, but also supporting the teammates that we have so that they can be prosperous, that they can find their purpose. They can be great problem solvers, but they can also be great providers for their family. And so my “Why” is to support that journey and that process that we’re going through right now.
Wow. Okay. Well, you look very young for having such a wise “Why,” but it’s good. You shortcut the process. I do agree that the journey is the destination, perhaps. It definitely is very important. And as an investment banker, I can testify that sometimes you saw businesses for people and then they fall into depression because they lost their identity. They didn’t know how to recreate the success that they were having along the way. And so that’s pretty awesome. So having said that, you are in a very highly evolving area, which is AI. You’ve worked very actively in AI. I mean, you do other stuff as well. But we talked about AI on our pre-call and I was very intrigued by the framework that you’ve developed around what you call the AI Operating System. So what is an AI Operating System and how do you get one in your company?
Sure. So I come from a supply chain background. I built nuclear submarines for the first five years of my life. And when you build for the government, you have to learn processes, but you also have to learn throughput. One of my favorite books of all time is The Goal by Eliyahu Goldratt. And it’s just a phenomenal book that teaches you about throughout and bottlenecks and relieving those bottlenecks to get to the next step so that you can ultimately achieve the profit. So with AI, we’ve been at this for eight years now. We’ve been developing a process and a strategy of how to implement AI into businesses.
What we’ve discovered is that CEOs are very scared of deploying something new, something that they don’t know and something that their friends or CEO friends are not doing the same way that they are. It was very easy to say, let’s move to the cloud because it had such a promising effect on the company that they could work from anywhere. The cloud would store all the data, they wouldn’t have to worry about servers going down. And all of their friends are moving to the clouds, all the other CEOs are moving to the cloud. So it was a transition that I think a lot of organizations took upon, whether it was Microsoft or Amazon, it didn’t matter. The movement to the cloud mattered. From our standpoint, AI is not so simple. AI becomes a very individualistic goal. It is something that your IP or your company has this fingerprint around, and you’re trying to AI that, which means it’s something different from your friends. It’s different from other companies. It’s a different organization.
And because everybody’s fingerprint is different, everybody’s adoption of AI is going to be different. But we’ve created a process of a workflow that you go through, we call it, you start with exploration, which is free, where you come through us and you try to figure out exactly what you’re trying to solve for. We then go through what we call product strategy and engineering discovery. And what that specifically does is diagnose what the problem is inside of the company and what the return on investment would be if we solve that problem. Now, I’ve been kind of making fun of Deloitte and Accenture for the last year because those two companies have this strategy session as well. It costs a million dollars, and they have great success with it. I’m not touting them for their success because a lot of companies see the value in hiring those types of consultants. What I am kind of knocking on is that to find value with AI should not take more than one or two months. The return on investments that we’re seeing are in the seven figures or even eight figures with a $200,000 investment. And AI changes the game for that initial discovery phase for what I call the strategy sessions. Because within a month, we should not only be able to determine what the ROI is, we should be very accurate with that. Because when AI is deployed properly, it either decreases costs or increased revenues. And we’ve seen that two dozen times in a row at this point. And we have the track record to prove that.
But the process should point out that once you solve for the KPIs, or once you’re trying to determine what the ROI is of this investment, it is now a system that you put in place to develop or implement that solution. It is no longer a what if, let’s test these three avenues. AI allows you to prototype, experiment, and deploy much quicker to get those results. So we like to say that every project we’ve worked on in the last, I would say, six to seven months has been less than four months of effort to get to a production release. We don’t build MVPs anymore, we don’t build prototypes, we build first production releases that get people into the system, working with it, testing it, and most importantly, seeing what the return on investment truly is based on the estimate. And if we achieve that return on investment, well, there’s more money to be had to keep building AI solutions. So everybody wins.
Okay, so let’s unpack this. So what does it mean to find the ROI? Give me an example of a project that you had kind of a hypothesis or an initial idea, and then you tested it and it showed an ROI and then you scaled it up. What does it look like?
Sure. So we had a consulting firm that worked for legal, it was a legal consulting firm. They help lawyers and paralegals determine what documentation and what information went into whether it’s prison reports or people that were sentenced to prison. We were able to double the revenues of that company and reduce the costs by about 90%. Now, when that happens, you’re basically allowing individuals to work the same way they did before. Nobody was fired. Everybody’s still supporting the initial goal, but they’re creating more revenue with the efforts they’re putting in. They’re also doubling down on what they’re good at, and they’re removing their waste. So, when an AI solution comes in, it allows all the consultants on the team, or a lot of the people that are doing the research to start getting this energy around what a new process looks like when built upon AI. And so this specific company, I mean, they have seen great success from not just the product itself, but from the time it takes to build the report.
Okay. So can you be more specific? What is it that they do differently with these reports? And how did you cut the cost by 90% if you kept everyone on the payroll? How is that even possible? What kind of costs could you eliminate?
Yeah, so the process that works very well with AI is when you’re looking at research or you’re looking at information of how a person is just looking at thesis paper or college kid looking through a thesis paper, it takes a lot of time to gather all of the links and the resources needed to write that thesis paper. And so let’s say it takes 10 hours to gather the information, another 10 hours or five hours to start writing that outline, then another few hours to take that outline and turn it into segments. And then finally, the writing of the thesis paper is your accumulation of all of that knowledge into one final paper. All of those steps can now be almost replaced by AI, except for the final writing piece. That piece in which the individual needs to take the knowledge that they’ve retrieved and make something new out of it.
That is where AI does not have a great success factor. It hallucinates. It doesn’t know what the human is trying to draw out of the research. And that’s where humans are so good. We can connect dots that don’t seemingly need to be connected, and we can make that connection. I mean, Malcolm Gladwell is phenomenal at connecting two unknown dots and then making you believe that that’s the truth. I’m not saying that he’s falsifying it, but he’s doing a great job at analogies and summarizing how things relate to each other. And humans are really good at that. But the cost of research, the cost of outlining, the cost of brief, that goes to the AI. So you’re reducing the cost of needing to do that aspect of it. All of the employees are still doing the human aspect of it, which is reporting the information necessary back to the client or the legal client and saying, this is what we found.
This is what the research told us. And instead of taking 20, 30, 40 hours to do that, you can do that in one hour. And so, you’re creating revenue, and the cost of performing those reports just goes from 20 hours or 30 hours down to one. More importantly, you can do 10, 20, 30, 40 reports where you used to be able to do two a week. And so that’s how the costs go down. It’s not that it’s like fabricated costs. Those costs would have existed if you got 40 on your desk, but they don’t exist because you have time to take on 40 new reports that you never did before.
Yeah. So, so essentially the cost per project goes down by 90%.
That’s right.
The actual cost of the company doesn’t, it stays the same perhaps.
That’s right.
And the revenue increases dramatically.
That’s right. I mean, cost is always a determination of it’s a ratio. COGS or operation expense, it’s always a ratio of revenue. So as you increase your revenue, as a team draws in more, you’re moving the bottleneck from the operations to the very end, which is the sales. And so, if sales is the limiting factor of how many reports you can do, well, no longer is that the case because the number of reports you can do, the throughput is much higher. So you can increase sales and have the same amount of throughput or increased throughput.
Yeah, okay, that makes sense. So that’s a really great way as a certain processes, AI can essentially streamline it, save a lot of time and let people do the stuff that only people can do for now, which is synthesizing, perhaps, certain concepts. So what are the types of AI solutions that you can deploy? So this is definitely creating research is one type. In our pre-call, we talked about AI agents, we talked about orchestration. So what kind of approaches there are big picture for AI deployment that someone could consider.
Yeah, there’s really three areas and they build on each other of where AI is going. The third one is not talked about much and we’ll get there in a second. But the first one is every company has a cadence, an IP, a knowledge base, a place in which they spend most of their time, whether it’s on Google Drive, sharing documents, trading information back and forth, communicating in Slack, all of that creates what we call culture, company culture. And Microsoft’s culture is very different than HubSpot’s culture. In fact, HubSpot has a glossary of three letter abbreviations that I don’t even know what 90% of them mean. But if you work at HubSpot, you surely do. And there are many cadences and there’s many cultures that have differences between their competitors. And what’s important about that is that it’s documentable, it’s proceduralized, and it’s also very, if you get hired into one and you move to another, you might not fit, even though you’re in the same industry. And what I’m trying to point out is that that culture and that cadence is an IP.
And every company has one, and they’ve been drilling for years and years and years, what they’re very specifically good at. They’re good at customer service. They’re maybe the cheapest price out there. Maybe they’re very good at how they relate to their clients. Maybe they go on site and they do certain consulting things. Maybe it’s a SAS product or maybe it’s a dropshipping and they’re the best dropshippers because they have two day delivery. Each one of those matters and that is why a knowledge base is the first place that AI should be built for every single one of these companies. When Amazon heard about large language models, guess what they did?
They built an internal large language model that only Amazon employees can use so that when you’re writing an email, you can say, what are the 900 emails that were similar to the one I’m trying to write internally for Amazon? Not around the world, not competitors, not other SaaS products. Internally on Amazon, what were the 900 emails related to how I wanted to ask for a new hire to get a promotion? That’s a very Amazon way of asking. They have a different promotion plan than most companies do. So to train that knowledge base on your internal IP and your company is the first step that every company should be doing. Now, Co-Pilot and Google Workspace are trying to enter into this space, and I would still argue that if you’re really interested in building AI, you need a consulting firm to come in and help you organize this the right way, because otherwise you’re just going to be what Google wants or what Microsoft wants. But that is 100% the first step. Once you have that knowledge base secured, you now can go to the second aspect, which is the agents.
You can deploy agents to do things like chat. You can deploy them to do invoice reconciliation. Or you can do, which our favorite is, workflow automation. When somebody passes documents from one person to the next, there’s an opportunity to reduce that workflow and either approve it or validate it or disapprove it or not allow it, whatever word you want to use to reduce the back and forth paperwork that humans have inside of companies. So agents can be deployed, they can have specific tasks, they can perform different functions, and that becomes your second introduction to AI. And it doesn’t work unless you have a knowledge base to relate back to. Like if you just deployed an agent, it’s not going to know how to act or how to perform or when to stop or when to misbehave or when to not behave or behave. So that agent thing becomes your second wave. Now the third one is where we’re heading. And that is when companies communicate to other companies through a large language model or through what we’re calling natural language.
No longer are APIs going to be the plumbing of the internet. Natural language is going to communicate between two pieces of software. So not just engineers can ask a question, but anybody in the organization can ask a question about their software. What is the invoice related to? How many orders are coming on plane 72 so I can get the shipments out the door? What is my cadence for understanding the exact processes of what I’m trying to do right now? What are the steps needed for me to do process A, B, and C? That is when I have to communicate with software, other systems, or even competitors. And as a product manager, designer, finance, HR, or developer, natural language or English should be the code of this plumbing line. And that’s what everybody’s building. That’s where we’re heading. That’s when a phone communicates to a laptop. That’s when your wife texts you, pick your kid up at six o’clock. And she doesn’t tell you the details, but it understands your calendar. It understands that every six o’clock ninja class happens and you need to go pick your kid up.
These things are coming down the pipeline and they’re coming fast and that only happens when a knowledge base is set up for either yourself or your company. Agents are deployed, which we’re calling MCP Service, but like basically these places are secure for agents to ask questions on your behalf and thirdly, for it to go outside the walled garden of your personal data and retrieve information for you.
Okay, so I’m trying to relate this to the use of ChatGPT, and maybe that’s not a good way to do it. But I’ve got a ChatGPT teams subscription, which is supposedly private. So what I do is, ChatGPT knows about me, knows what I do, knows the kinds of clients I’m working with, and understands some of the products I have. And then I basically ask it to do certain things. So I wonder if that’s similar to deploying agents. So now it knows my knowledge base. Maybe it’s not completely built out like it should be, but at some level it knows. So I can ask questions and then even create workflows. But the third step is to communicate with software, other competitors, and so on. I don’t understand exactly how that works. So what is the step between agents and this natural language communication? What is the missing step that needs to be breached to get there?
Sure, so in relationship to ChatGPT, the first thing that you’re doing is creating a knowledge base of things you know so that ChatGPT when it prompts back to you and retrieves the answers for you, it’s retrieving in context that you understand. So while you have a mini knowledge base and you’re putting information into a project or to Microsoft Co-Pilot, that knowledge base is what’s informing ChatGPT of the answer it should be giving. That’s step one. Step two is the agent that you are, like a web agent. When it crawls the Internet, that is an agent going out to crawl the web, looking at the first eight links of Google, grabbing information back and bringing that to you to say, hey, I searched the Internet for you and I found this answer.
That’s an agent. But you can also imagine an agent with your Excel documents. Maybe you put in a number of shipments every single month into column B2. Well, an agent can do that for you after the shipment is retrieved from your invoicing documents. So because it has a knowledge base and because it has a knowledge base and because it has information about your shipping reports and your books, you can write an agent to say every time we retrieve our shipments, go up to this Excel sheet and fill in the correct number. But it’s not just an API, it’s smart enough to know that a half a shipment matters and it should be 7.5. It’s smart enough to know that if you have a negative shipment, well, that also means that the stuff is on the way, it just hasn’t been fulfilled yet.
It’s basically an automation that I create that will do repetitive tasks.
That’s right, it’s automation to create, and any agent can do, there can be small agents, they can be teeny tiny to do something simple, and they could be massive agents, like a chatbot that answers customer queries. Those are the level of agents. And the third one is going outside your walled garden. The best example that I’ve given repeatedly is you’re standing on the corner, you’re at your house and you’re ready to go to the airport. You should be able to say, I would like a ride to the airport and I would like to stop and get maybe some deodorant or maybe you want to get a sandwich on the way to the airport. And I would also like to get there a little bit early so I can have a presentation. I can do a phone call from the lounge.
Now to do that, two things need to be happening that don’t happen right now. One is security and privacy. The other one is the ability for these agents to send information out discreetly on your behalf. In this scenario, the ultimate goal is that agent goes out and crawls Uber, locates an Uber car for you and brings it to your house at the specific time needed for you to go to the airport. The second one would be to understand the mapping system between your house and the airport to figure to stop at CVS and maybe just stop at a sandwich. Most importantly, a sandwich shop that you’ve been to before that you like, that you’ve said before you’ve enjoyed. And then lastly, to understand your calendar, gather the meeting that you’re trying to be in, make sure that you’re at the airport with plenty of time for you to get through security and to your meeting. That requires your agents to communicate with other servers that you don’t have privy to or information to, or maybe you have a login to Delta and maybe you have a login to get to their security and make sure you’re validated. But these agents need to have security when they go out, just like an API call has a secret key.
Those agents are going to have to go out and on your behalf, act on what you want them to do and securely keep your information away from prying marketers that then intercept that. And all of a sudden, Fluffy’s car service shows to pick you up. And if Fluffy’s car service shows up and you were expecting Uber, you’re not going to be a happy client. But what happened there is Fluffy’s car service decided that they had a better marketing team and they were able to intercept these agents and deliver cars all around the world. And that’s what can start happening if we don’t keep these things secure when they go out and make the requests on our behalf.
So that sounds like a lot of complexity. How far are we from these super agents that can use my personal information?
So on Google, it’s already here. It’s unfortunate that iPhone has such a large footprint in America because I don’t think people are realizing the advancements Google has made in the last two months on their Gemini platform. I, right now, can book a trip, I can play a song, I can just have Spotify playing in the background, which seem relatively general, but when you have a large language model that understands your calendar, Gmail, your text messages, and also your internal drive, like my personal drive, it knows information about me while I’m requesting in my voice assistant. And so Google has made these great advancements with what they call Gems in the last month. I imagine that we’re gonna see an Uber Gem, an Airbnb Gem, all of that is going to come into this Google ecosystem. And they’re three years ahead of Apple at this point, in my opinion.
Wow, that’s pretty crazy. Now, what about the security aspect of it? Because obviously, I mean, more and more, I tried to download something, it always asked me to confirm that I trust this company, it can use all my information, it has access to my email and all that stuff. And it’s very scary. Sometimes I say, no way, I’m not going to do that. It’s just feels too intimidating to do this. And then plus people are concerned about government listening in. How can this thing can grow without people being overly exposed to their information?
Yeah, so recently, we’ve always had this problem and we haven’t been able to name it, just like we weren’t able to name what Retrieval-Augmented Generation was. There was always these things that we were doing inside of our company, as we’re AI consultants, we build these models all the time. And we were doing exactly this, we just didn’t have a name for it. And recently, Claude, I believe, was the first to coin the phrase Model Context Protocol. It’s a standardized way for AI applications, which basically like think of large language models, that to interact with external tools and data sources. And they act as like a universal connector. So think of like a USB-C and a USB-C port. You have the USB-C port and you want to plug into Uber and Airbnb and the receiving end, and you need a USB-C receiver to plug into. Well, it secures your privacy. It secures your information.
It secures who you are and kind of tokenizes you. And so, then every time you want to use an Uber request, the large language model can use that Uber request securely to retrieve your information about your Uber and back to you. So it’s kind of the new plumbing that’s happening across the internet. That MTP server is becoming the terminology that we’re using of how that all works. It’s the plumbing of APIs for large language models.
Yeah. And so all I have to do is trust Google who owns all these products?
No, I’ve been speaking about this. I hope that companies like Perplexity or Claude are, they have the ability to get onto hardware. And so, right now, Google and Apple have a massive advantage because they have the hardware and the voice assistance built into those devices. So they, for sure, have a duopoly around where most people are going to be sharing their information. And Google already has been doing it for me for a few months now. I hope that Perplexity and Claude and even OpenAI comes out with hardware that I can select that piece of hardware where I maybe own end-to-end encryption about the device in my large language model so that I can control the data security. So that little checkbox that you’re saying when you ask for your calendar to be shared, maybe that checkbox is something that you truly can protect against and Google doesn’t just know about it anyways.
Yeah. I mean, even when companies request me to sign in through Google, I always think, okay, what is it going to leak from Google to this company? I’m giving some permission. Do I really understand exactly what permission I’m giving? It’s just a very uncomfortable situation.
Exactly. And I think that hopefully can be reversed because if you have all the information necessary on your phone through the large language model, hopefully we can call apps and retrieve like if I wanted to buy a toaster, I don’t need to go to Amazon to search toaster to find one. I should be able to search my voice assistant for the best toaster, decide that it’s on Amazon and click purchase and the agent should take care of the rest from there.
Okay. Well, brave new world. Definitely very exciting. So I love this clarity around building out the AI. So first, the knowledge base. Create your own knowledge base and train your own AI, and then deploy agents. So essentially automate all your repeatable processes so that you can save all the energy to bigger tasks. And then maybe leverage something like Gemini that will coordinate multiple of these agents, super agent kind of thing. Maybe I misunderstand it, but it feels to me that it’s kind of a coordination of multiple agents, it’s kind of the manager of the agent workforce that is going to be doing things for you and you just give them instructions. It’s essentially a delegate to this personal integrator, whatever you want to call it.
Exactly. No, you got it. That’s the process.
That’s, that’s awesome. Okay. So our time is coming to the end here. Let me ask you one more question here. So as an entrepreneur, it was very interesting how your “Why” evolved. You wanted this tallest building with the logo and now you really want to journey and to enjoy working with the great people and working on exciting projects. What do you feel is the most important question any entrepreneur should be asking themselves?
I think the obvious one to me now is the vocation. Like chasing money is one thing. But if you truly like what you do, you shouldn’t have to worry about the money because you should be able to find enough work in that area to be successful. So, there’s a lot of entrepreneur advice out there of chasing dropshipping or chasing different areas that are the hot topic of the day. And it seems like a quick way to make money. But if you truly can’t see yourself being there for 10, 20 years, it might not be worth the effort to go into a market like that. And I also would say the same thing about TAM, like if your total addressable market is just not that big, and it’s a little tiny market, it might not be advantageous for you to put all your energy into that.
I would look at your next 10 years or 20 years and figure out where do you really enjoy being, who do you enjoy talking to, who do you enjoy working with, and what type of work do you wanna do in 10 years, and then start learning in that direction. Because if you work the other way and chase the money, you’ll end up in an industry with all these skills that might not be fruitful for a long period of time. So I truly love what I do. So I’m in the camp of finding an area. I love consulting. I love being an apprentice. I love building products. And being a consultant is all three of those, especially an AI consultant. I think people should look at that first and figure out, all right, what would excite me in 10, 20 years? And is there a big enough market that I can make my million dollars in that area and work backwards and try to figure out how to learn to be in that vocation?
Yeah, I think it’s very smart, especially because if you were to choose for the money, then it’s gonna be really hard for you to be successful without not loving what you do.
Exactly right.
So it might not even work. I mean, maybe it’s not just the fleetingness of those areas. Maybe dropshipping is a fleeting thing. I don’t know. It’s a hype thing, but you can’t even get there without loving the stuff.
I agree.
Yeah, that’s cool. All right. Well, so Andrew, if people would like to build their own AI Operating System? Where can they find you and how can they get more information or get started?
Sure, so I’m on LinkedIn. I post once a day. You can message me there. I read all the comments. Andrew A-M-A-N-N, and my company is NineTwoThree. You can fill out a form if you’re interested in a project, but for the most part, following us is the best thing I can ask for.
Well, definitely go and follow Andrew Amann, check his posts and also get immersed in AI because it’s moving very fast and you’re gonna miss the boat if you don’t. And if you enjoyed this episode, make sure you follow us on YouTube and give us a review on Apple Podcasts and join next week when an exciting entrepreneur is going to bring a framework to us. So, thank you for coming, Andrew, and thanks for listening.