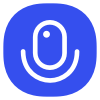
Sign up to save your podcasts
Or
The episode discuss recent advances in improving the capabilities of transformer-based natural language processing (NLP) models.
One article focuses on a novel approach called Mixtures of In-Context Learners (MoICL) that addresses memory limitations and improves classification accuracy by combining multiple in-context learners.
The other article explores the Buffer of Thoughts (BoT) approach which enhances reasoning abilities, and the use of filler tokens to enhance computational capabilities in complex problem solving.
These research areas aim to overcome challenges related to limited memory, reasoning abilities, and computational constraints in NLP models.
The episode discuss recent advances in improving the capabilities of transformer-based natural language processing (NLP) models.
One article focuses on a novel approach called Mixtures of In-Context Learners (MoICL) that addresses memory limitations and improves classification accuracy by combining multiple in-context learners.
The other article explores the Buffer of Thoughts (BoT) approach which enhances reasoning abilities, and the use of filler tokens to enhance computational capabilities in complex problem solving.
These research areas aim to overcome challenges related to limited memory, reasoning abilities, and computational constraints in NLP models.