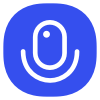
Sign up to save your podcasts
Or
This research addresses the challenge of catastrophic forgetting in large language models during continual learning, where adapting to new tasks degrades performance on old ones. To overcome this, the authors introduce a novel approach that utilizes adaptive singular value decomposition (SVD) to identify and preserve important knowledge while allowing flexible learning of new information. Their method dynamically determines task-specific low-rank parameter subspaces for updates, ensuring these updates remain orthogonal to critical directions learned from prior tasks. This constrained full fine-tuning technique achieves state-of-the-art results on various benchmarks, demonstrating effective knowledge retention and adaptation without increasing the model's parameter count or requiring storage of past gradients.
This research addresses the challenge of catastrophic forgetting in large language models during continual learning, where adapting to new tasks degrades performance on old ones. To overcome this, the authors introduce a novel approach that utilizes adaptive singular value decomposition (SVD) to identify and preserve important knowledge while allowing flexible learning of new information. Their method dynamically determines task-specific low-rank parameter subspaces for updates, ensuring these updates remain orthogonal to critical directions learned from prior tasks. This constrained full fine-tuning technique achieves state-of-the-art results on various benchmarks, demonstrating effective knowledge retention and adaptation without increasing the model's parameter count or requiring storage of past gradients.