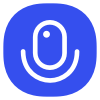
Sign up to save your podcasts
Or
The first source, a research paper from Arizona State University, explores the abilities of large language models (LLMs) to plan, using a benchmark called PlanBench. While LLMs have shown some improvement, they struggle with complex tasks. The paper highlights the emergence of a new model, o1, described as a Large Reasoning Model (LRM), which demonstrates better performance on PlanBench, but still falls short of robust, guaranteed solutions. The second source, an addendum to a previous Nature article, introduces AlphaChip, a deep reinforcement learning method developed by Google to generate chip layouts. This method has been successful in improving chip design, but its effectiveness is dependent on extensive pre-training and computational resources. The authors address misconceptions about the approach and emphasize its real-world applications, including its use in Google's Tensor Processing Unit (TPU).
The first source, a research paper from Arizona State University, explores the abilities of large language models (LLMs) to plan, using a benchmark called PlanBench. While LLMs have shown some improvement, they struggle with complex tasks. The paper highlights the emergence of a new model, o1, described as a Large Reasoning Model (LRM), which demonstrates better performance on PlanBench, but still falls short of robust, guaranteed solutions. The second source, an addendum to a previous Nature article, introduces AlphaChip, a deep reinforcement learning method developed by Google to generate chip layouts. This method has been successful in improving chip design, but its effectiveness is dependent on extensive pre-training and computational resources. The authors address misconceptions about the approach and emphasize its real-world applications, including its use in Google's Tensor Processing Unit (TPU).