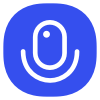
Sign up to save your podcasts
Or
In this episode, we explore the latest advancements in automated red teaming from OpenAI, presented in the paper "Diverse and Effective Red Teaming with Auto-generated Rewards and Multi-step Reinforcement Learning." Automated red teaming has become essential for discovering rare failures and generating challenging test cases for large language models (LLMs). This paper tackles a core challenge: how to ensure attacks are both diverse and effective.
We dive into their two-step approach:
Discover how this approach improves on previous methods by generating more varied and successful attacks, including prompt injection attacks and unsafe response prompts, paving the way for more robust AI models.
Paper: Beutel A, Xiao K, Heidecke J, Weng L "Diverse and Effective Red Teaming with Auto-generated Rewards and Multi-step Reinforcement Learning." (2024). OpenAI.com
Disclaimer: This podcast summary was generated using Google's NotebookLM AI. While the summary aims to provide an overview, it is recommended to refer to the original research preprint for a comprehensive understanding of the study and its findings.
In this episode, we explore the latest advancements in automated red teaming from OpenAI, presented in the paper "Diverse and Effective Red Teaming with Auto-generated Rewards and Multi-step Reinforcement Learning." Automated red teaming has become essential for discovering rare failures and generating challenging test cases for large language models (LLMs). This paper tackles a core challenge: how to ensure attacks are both diverse and effective.
We dive into their two-step approach:
Discover how this approach improves on previous methods by generating more varied and successful attacks, including prompt injection attacks and unsafe response prompts, paving the way for more robust AI models.
Paper: Beutel A, Xiao K, Heidecke J, Weng L "Diverse and Effective Red Teaming with Auto-generated Rewards and Multi-step Reinforcement Learning." (2024). OpenAI.com
Disclaimer: This podcast summary was generated using Google's NotebookLM AI. While the summary aims to provide an overview, it is recommended to refer to the original research preprint for a comprehensive understanding of the study and its findings.