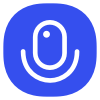
Sign up to save your podcasts
Or
This Amazon Science blog post introduces HalluMeasure, a novel approach for automatically detecting hallucinationsin large language model outputs. The method employs a three-pronged strategy combining claim-level evaluations, chain-of-thought reasoning, and the classification of hallucination error types. By decomposing LLM responses into individual claims and comparing them to relevant context, HalluMeasure aims to improve the accuracy of identifying incorrect assertions. Furthermore, it categorizes these errors into specific linguistic types, offering valuable insights for developers to refine their models. The described research presented at EMNLP 2024 demonstrates enhanced performance in hallucination detection compared to existing solutions.
This Amazon Science blog post introduces HalluMeasure, a novel approach for automatically detecting hallucinationsin large language model outputs. The method employs a three-pronged strategy combining claim-level evaluations, chain-of-thought reasoning, and the classification of hallucination error types. By decomposing LLM responses into individual claims and comparing them to relevant context, HalluMeasure aims to improve the accuracy of identifying incorrect assertions. Furthermore, it categorizes these errors into specific linguistic types, offering valuable insights for developers to refine their models. The described research presented at EMNLP 2024 demonstrates enhanced performance in hallucination detection compared to existing solutions.