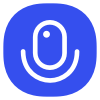
Sign up to save your podcasts
Or
We discuss a presentation and discussion on training language models (LMs) using distributed, or siloed, data, which is often proprietary and cannot be combined into a single dataset for joint training. The speaker highlights the importance of data for LM performance and the increasing trend of valuable data becoming proprietary, making traditional joint training approaches challenging. The presentation proposes a novel method, termed SILO Open LM, which adapts the Mixture-of-Experts (MoE) architecture and leverages "MoE-aware silo training" and "obtaining proxy data" to train LMs on isolated datasets and merge them into a single, general-purpose model. Experimental results comparing this approach to existing methods like weight merging and ensembling are presented, demonstrating significant performance gains on various benchmarks. The work also acknowledges limitations and open research questions, including improving performance on specialized tasks and scaling the approach to a larger number of datasets.
We discuss a presentation and discussion on training language models (LMs) using distributed, or siloed, data, which is often proprietary and cannot be combined into a single dataset for joint training. The speaker highlights the importance of data for LM performance and the increasing trend of valuable data becoming proprietary, making traditional joint training approaches challenging. The presentation proposes a novel method, termed SILO Open LM, which adapts the Mixture-of-Experts (MoE) architecture and leverages "MoE-aware silo training" and "obtaining proxy data" to train LMs on isolated datasets and merge them into a single, general-purpose model. Experimental results comparing this approach to existing methods like weight merging and ensembling are presented, demonstrating significant performance gains on various benchmarks. The work also acknowledges limitations and open research questions, including improving performance on specialized tasks and scaling the approach to a larger number of datasets.