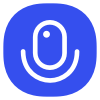
Sign up to save your podcasts
Or
This episode breaks down the 'Multi-token Prediction' research paper, which proposes a novel approach to training large language models (LLMs) called multi-token prediction, where the model learns to predict multiple future tokens at once, rather than just the next one. The authors argue that this method leads to improved sample efficiency, particularly for larger models. This means that LLMs trained with multi-token prediction can achieve similar performance levels with less data. Additionally, multi-token prediction enables self-speculative decoding, which can significantly speed up inference time. The paper provides experimental evidence supporting these claims across various benchmarks, including coding tasks and natural language processing tasks.
Audio : (Spotify) https://open.spotify.com/episode/2fxn61GdH3PrJoxdcIPk77?si=dREu4yTpTWKYyfEj9p86dA
Paper: https://arxiv.org/pdf/2404.19737
This episode breaks down the 'Multi-token Prediction' research paper, which proposes a novel approach to training large language models (LLMs) called multi-token prediction, where the model learns to predict multiple future tokens at once, rather than just the next one. The authors argue that this method leads to improved sample efficiency, particularly for larger models. This means that LLMs trained with multi-token prediction can achieve similar performance levels with less data. Additionally, multi-token prediction enables self-speculative decoding, which can significantly speed up inference time. The paper provides experimental evidence supporting these claims across various benchmarks, including coding tasks and natural language processing tasks.
Audio : (Spotify) https://open.spotify.com/episode/2fxn61GdH3PrJoxdcIPk77?si=dREu4yTpTWKYyfEj9p86dA
Paper: https://arxiv.org/pdf/2404.19737