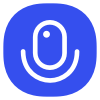
Sign up to save your podcasts
Or
This paper introduces DICE, a novel method for enhancing large language model (LLM) alignment with human preferences by bootstrapping using the implicit reward model generated through Direct Preference Optimization (DPO). Unlike traditional approaches that rely on external feedback or explicitly trained reward models, DICE leverages the reward signal inherent in a DPO-tuned model to create new preference data. To improve the quality of this self-generated data and prevent issues like favoring overly long responses, the method incorporates length-regularized reward shaping and experience replay of the initial human preference data. Empirical results demonstrate that this iterative self-alignment process significantly boosts the model's performance on standard benchmarks.
This paper introduces DICE, a novel method for enhancing large language model (LLM) alignment with human preferences by bootstrapping using the implicit reward model generated through Direct Preference Optimization (DPO). Unlike traditional approaches that rely on external feedback or explicitly trained reward models, DICE leverages the reward signal inherent in a DPO-tuned model to create new preference data. To improve the quality of this self-generated data and prevent issues like favoring overly long responses, the method incorporates length-regularized reward shaping and experience replay of the initial human preference data. Empirical results demonstrate that this iterative self-alignment process significantly boosts the model's performance on standard benchmarks.