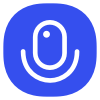
Sign up to save your podcasts
Or
Breandan Considine is a PhD student at the School of Computer Science at McGill University, under the supervision of Jin Guo and Xujie Si). There, he is building tools to help developers locate and reason about software artifacts, by learning to read and write code.
Disclaimer: when talking to people in this podcast I try to sometimes invite guests who share different inside views about existential risk from AI so that everyone in the AI community can talk to each other more and coordinate more effectively. Breandan is overall much more optimistic about the potential risks from AI than a lot of people working in AI Alignement research, but I think he is quite articulate in his position, even though I disagree with many of his assumptions. I believe his point of view is important to understand what software engineers and Symbolic reasoning researchers think of deep learning progress.
Transcript: https://theinsideview.ai/breandan
Youtube: https://youtu.be/Bo6jO7MIsIU
Host: https://twitter.com/MichaelTrazzi
Breandan: https://twitter.com/breandan
OUTLINE
(00:00) Introduction
(01:16) Do We Need Symbolic Reasoning to Get To AGI?
(05:41) Merging Symbolic Reasoning & Deep Learning for Powerful AI Systems
(10:57) Blending Symbolic Reasoning & Machine Learning Elegantly
(15:15) Enhancing Abstractions & Safety in Machine Learning
(21:28) AlphaTensor's Applicability May Be Overstated
(24:31) AI Safety, Alignment & Encoding Human Values in Code
(29:56) Code Research: Moral, Information & Software Aspects
(34:17) Automating Programming & Self-Improving AI
(36:25) Debunking AI "Monsters" & World Domination Complexities
(43:22) Neural Networks: Limits, Scaling Laws & Computation Challenges
(59:54) Real-world Software Development vs. Competitive Programming
(1:02:59) Measuring Programmer Productivity & Evaluating AI-generated Code
(1:06:09) Unintended Consequences, Reward Misspecification & AI-Human Symbiosis
(1:16:59) AI's Superior Intelligence: Impact, Self-Improvement & Turing Test Predictions
(1:23:52) AI Scaling, Optimization Trade-offs & Economic Viability
(1:29:02) Metrics, Misspecifications & AI's Rich Task Diversity
(1:30:48) Federated Learning & AI Agent Speed Comparisons
(1:32:56) AI Timelines, Regulation & Self-Regulating Systems
2
11 ratings
Breandan Considine is a PhD student at the School of Computer Science at McGill University, under the supervision of Jin Guo and Xujie Si). There, he is building tools to help developers locate and reason about software artifacts, by learning to read and write code.
Disclaimer: when talking to people in this podcast I try to sometimes invite guests who share different inside views about existential risk from AI so that everyone in the AI community can talk to each other more and coordinate more effectively. Breandan is overall much more optimistic about the potential risks from AI than a lot of people working in AI Alignement research, but I think he is quite articulate in his position, even though I disagree with many of his assumptions. I believe his point of view is important to understand what software engineers and Symbolic reasoning researchers think of deep learning progress.
Transcript: https://theinsideview.ai/breandan
Youtube: https://youtu.be/Bo6jO7MIsIU
Host: https://twitter.com/MichaelTrazzi
Breandan: https://twitter.com/breandan
OUTLINE
(00:00) Introduction
(01:16) Do We Need Symbolic Reasoning to Get To AGI?
(05:41) Merging Symbolic Reasoning & Deep Learning for Powerful AI Systems
(10:57) Blending Symbolic Reasoning & Machine Learning Elegantly
(15:15) Enhancing Abstractions & Safety in Machine Learning
(21:28) AlphaTensor's Applicability May Be Overstated
(24:31) AI Safety, Alignment & Encoding Human Values in Code
(29:56) Code Research: Moral, Information & Software Aspects
(34:17) Automating Programming & Self-Improving AI
(36:25) Debunking AI "Monsters" & World Domination Complexities
(43:22) Neural Networks: Limits, Scaling Laws & Computation Challenges
(59:54) Real-world Software Development vs. Competitive Programming
(1:02:59) Measuring Programmer Productivity & Evaluating AI-generated Code
(1:06:09) Unintended Consequences, Reward Misspecification & AI-Human Symbiosis
(1:16:59) AI's Superior Intelligence: Impact, Self-Improvement & Turing Test Predictions
(1:23:52) AI Scaling, Optimization Trade-offs & Economic Viability
(1:29:02) Metrics, Misspecifications & AI's Rich Task Diversity
(1:30:48) Federated Learning & AI Agent Speed Comparisons
(1:32:56) AI Timelines, Regulation & Self-Regulating Systems