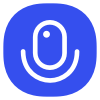
Sign up to save your podcasts
Or
Making a data pipeline fit for machine learning use cases requires more than just additional data monitoring. Furthermore, bringing machine learning into production has traditionally required a lot of manual setup and configuration, even for toy ML pipelines. These manual methods are not reproducible, don’t autoscale, require significant technical expertise, and are error-prone. Among other things, this episode will go over MLOps, a set of practices aiming to deploy and maintain machine learning models in production reliably and efficiently.
Making a data pipeline fit for machine learning use cases requires more than just additional data monitoring. Furthermore, bringing machine learning into production has traditionally required a lot of manual setup and configuration, even for toy ML pipelines. These manual methods are not reproducible, don’t autoscale, require significant technical expertise, and are error-prone. Among other things, this episode will go over MLOps, a set of practices aiming to deploy and maintain machine learning models in production reliably and efficiently.