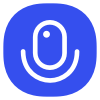
Sign up to save your podcasts
Or
We examine how biases in large language models (LLMs) can be understood and addressed from a causal perspective, specifically identifying training data and input prompts as key confounders contributing to biased outputs. The researchers propose Causality-Aware Alignment (CAA), a novel method leveraging reinforcement learning with interventional feedback derived from a reward model acting as an instrumental variable. By analyzing the difference in outputs between an initial LLM and an intervened one, CAA generates sample weights to guide RL finetuning, effectively reducing biases in generated text as demonstrated in experiments across various tasks. This approach highlights the importance of considering causal relationships in LLM alignment to achieve less biased and safer outputs.
We examine how biases in large language models (LLMs) can be understood and addressed from a causal perspective, specifically identifying training data and input prompts as key confounders contributing to biased outputs. The researchers propose Causality-Aware Alignment (CAA), a novel method leveraging reinforcement learning with interventional feedback derived from a reward model acting as an instrumental variable. By analyzing the difference in outputs between an initial LLM and an intervened one, CAA generates sample weights to guide RL finetuning, effectively reducing biases in generated text as demonstrated in experiments across various tasks. This approach highlights the importance of considering causal relationships in LLM alignment to achieve less biased and safer outputs.