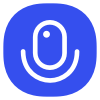
Sign up to save your podcasts
Or
[DISCLAIMER] - For the full visual experience, we recommend you tune in through our YouTube channel to see the presented slides.
Try datamol.io - the open source toolkit that simplifies molecular processing and featurization workflows for machine learning scientists working in drug discovery: https://datamol.io/
If you enjoyed this talk, consider joining the Molecular Modeling and Drug Discovery (M2D2) talks live.
Also, consider joining the M2D2 Slack.
Abstract: Developing novel bioactive molecules is time-consuming, costly and rarely successful. As a mitigation strategy, we utilize, for the first time, cellular morphology to directly guide the de novo design of small molecules. We trained a conditional generative adversarial network on a set of 30 000 compounds using their cell painting morphological profiles as conditioning. Our model was able to learn chemistry-morphology relationships and influence the generated chemical space according to the morphological profile. We provide evidence for the targeted generation of known agonists when conditioning on gene overexpression profiles, even though no information on biological targets was used during training. Based on a target-agnostic readout, our approach facilitates knowledge transfer between biological pathways and can be used to design bioactives for many targets under one unified framework. Prospective application of this proof-of-concept to larger chemical spaces promises great potential for hit generation in drug and phytopharmaceutical discovery and chemical safety.
Speaker: Paula A. Marin Zapata
Twitter - Prudencio
Twitter - Therence
Twitter - Jonny
Twitter - datamol.io
[DISCLAIMER] - For the full visual experience, we recommend you tune in through our YouTube channel to see the presented slides.
Try datamol.io - the open source toolkit that simplifies molecular processing and featurization workflows for machine learning scientists working in drug discovery: https://datamol.io/
If you enjoyed this talk, consider joining the Molecular Modeling and Drug Discovery (M2D2) talks live.
Also, consider joining the M2D2 Slack.
Abstract: Developing novel bioactive molecules is time-consuming, costly and rarely successful. As a mitigation strategy, we utilize, for the first time, cellular morphology to directly guide the de novo design of small molecules. We trained a conditional generative adversarial network on a set of 30 000 compounds using their cell painting morphological profiles as conditioning. Our model was able to learn chemistry-morphology relationships and influence the generated chemical space according to the morphological profile. We provide evidence for the targeted generation of known agonists when conditioning on gene overexpression profiles, even though no information on biological targets was used during training. Based on a target-agnostic readout, our approach facilitates knowledge transfer between biological pathways and can be used to design bioactives for many targets under one unified framework. Prospective application of this proof-of-concept to larger chemical spaces promises great potential for hit generation in drug and phytopharmaceutical discovery and chemical safety.
Speaker: Paula A. Marin Zapata
Twitter - Prudencio
Twitter - Therence
Twitter - Jonny
Twitter - datamol.io