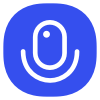
Sign up to save your podcasts
Or
CHAPTER 7: Refine Your Onboarding Success Criteria
If you do not know where you are going, every road will get you nowhere.
- Henry A. Kissinger
Google Maps can be quite a marvel.
The vast majority of the time, it gets us where we need to go without having to think about it. We just plug in our destination, and boom, we’re there. So many of us have become so reliant on it that when it fails, everything goes awry.
I experienced this firsthand with my wife Joanna on a road trip from Toronto to Montreal. Unfamiliar with the streets of Montreal, I relied heavily on Google Maps to find our hotel. Low and behold, my phone lost signal after exiting an underground tunnel, and I received that unbearable error message, “GPS lost signal.”
We ended up driving over a bridge that led to the US-Canada border. That’s not so bad if we both had our Canadian passports, but we didn’t!
Immediately, we both started freaking out. How are we supposed to explain to the Canadian Border Agents that we’re not illegal immigrants trying to sneak into the United States of America, a.k.a. the land of the free?
Of course, we completely overreacted. The Canadian Border Services Agency officer we spoke with was understanding. After showing him our Ontario driver’s license and explaining the Google Maps failure, he laughed it off. All we had to do was exit on a ramp that led us back to where we needed to go.
This is a harmless story, where not knowing your destination can lead you down the wrong path. But it doesn’t always end well, especially with the cross-functional, highly-visible effort of improving user onboarding.
By now, you should have a clear picture of what your users’ desired outcomes are along with the functional, emotional, and social jobs they are hiring your product for. You should also now know the four progress-making forces that influence the decision to adopt or drop the product.
The next step is to define what success looks like for your user onboarding experience. There are two moments that matter the most to measure onboarding success:
These two success criteria are tied to key milestones in the user onboarding: the Moment of Value Experience and the Moment of Value Adoption.
Success Criteria #1: The First Strike
The first onboarding success criteria is helping users achieve their desired outcome, or Customer Job, as quickly as possible.
In Wes Bush’s Bowling Alley framework, this event is called the “First Strike.” In 10-pin bowling, this happens when all ten pins are knocked down with a bowling ball. (We’ll go in-depth into the Bowling Alley framework in the next chapter.)
In user onboarding, the First Strike is a necessary product action users must take to accomplish their desired outcome or Customer Job. Let me give some examples.
For some companies, this First Strike isn’t so obvious. Think about Facebook. What’s their First Strike? Is it a user liking, sharing, or commenting on posts?
Since those actions require users to have a few Facebook friends, the most important product action is to add a friend. That’s why Facebook prompts new users to connect with friends as quickly as possible. This happens early on in their onboarding process.
For “all-in-one” products that solve many problems, recognizing the First Strike is even more tricky. Imagine identifying the First Strike for a Swiss Army knife. Is it the knife, pen, screwdriver, compass, scissors, saw blade, fire starter, or laser pointer?
Many all-in-one B2B SaaS products can essentially be considered digital Swiss Army knives. How do you determine the First Strike in these cases?
This is where context and segment matter. Depending on the context of use and the segment, there could be different First Strikes. For example, users can accomplish a lot with Intercom:
So, what is Intercom’s First Strike?
It’s hard to decipher at first glance. But by segmenting Intercom’s users, you learn that users hire Intercom for three core Customer Jobs, all aligning with their three product lines:
As you can see, the First Strike is closely tied to your product’s Customer Job (or Jobs, for more complex products). It’s the first measure of success that new users are on the right track.
Success Criteria #2: The Product Adoption Indicator
The second measure of success is the moment users start using a product consistently.
One of the end goals of onboarding is helping users embrace new habits with a product. Habit-forming user onboarding requires users to experience the value of a product more than once. If new users have used the product enough times, they’re more likely to continue with it going forward.
For Slack, a team is not successfully onboarded until they’ve sent not one, not 10, but 2,000 messages. It’s at this threshold where they’ve found the teams who are likely to continue using it.
Since this onboarding success metric is a leading indicator of user retention and product adoption, I call this the Product Adoption Indicator, or PAI for short. It’s an early but strong signal that users are likely to continue using a product going forward. They have embraced the product and are very unlikely to return to their old habits.
This concept is not new—others call this the “magic number.” Here are some well-known examples:
Notice that the PAIs for each of these products are closely tied to retention.
Once users reach this point, you’ve set them up for success. They’re now ready to take the next step in the product’s customer journey. They’ve completed the initial loop of the user onboarding process: they’ve perceived, experienced, and adopted your product for the first time.
Good PAIs have a few common characteristics:
The Five Steps To Determine Your PAI
There are five steps to establishing a company’s PAI.
I’ll be using Whatsapp as an example for this exercise. All data used below are fictional and used for instructional purposes only.
1. Get a baseline measurement of your retention.
As previously discussed, one result from good onboarding is a lift in retention rate. Before making any changes to the user onboarding, you’ll want to first define what retention looks like.
One way to do this is to perform a cohort analysis using a retention chart and curve. This reflects the percentage of users who come back and perform any action with a product several days or weeks after signing up.
With analytics tools like Mixpanel or Amplitude, you should have readily available reports to visualize a product’s retention curve. You could also calculate this metric by manually using Excel or Google Sheets.
For example, let’s say 12,481 users downloaded your app on January 1, and you want to calculate the retention rate for the first seven days. You need to track:
Next, you need to divide the number of returning users by the total number of users, then multiply by 100.
Out of the 12,481 users who started on January 1, only 3,506 end up returning on January 2.
Day 1 retention is: (3,506/12,481)*100 = 28.1%.
Repeat the same process for the remaining days to calculate your retention table:
Plot this on a chart, with the days on the horizontal axis and the retention rates are on the vertical axis. By doing this, you can calculate the baseline retention curve for your current user onboarding experience.
2. Create a PAI hypothesis.
Once you’ve identified the baseline retention curve, it’s time to create a hypothesis about your retention indicator. It should look something like this:
If new users perform at least X number of [the one key product action] during the first Y days/weeks of signing up, they’re more likely to continue using our product after Z days/weeks.
To create your own, let’s back up to break it down:
The final hypothesis for this example is:
If new Whatsapp users send at least three messages on the day of signing up, they’re more likely to continue using it after 21 days.
3. Gather data to validate your hypothesis.
What we’re looking for here is to maximize the overlap between the following two segments:
Another way to visualize this is by using a Venn diagram. The idea is to maximize the surface area between the segment of users who continue using Whatsapp after 21 days and the segment of users who have sent at least X messages on the first day of signing up.
If you take a look at the segment of users who have sent at least nine messages on the day of signing up, many might continue to use Whatsapp after 21 days.
But there could be quite a few retained Whatsapp users who don’t meet that number. In this case, the threshold for the PAI is too high. This is because we want to identify the minimum number of messages that also correspond to users who continue to use Whatsapp after 21 days.
On the other end, most users who send at least one message on the day of signing up did not continue using Whatsapp after 21 days. In this case, the threshold is too low.
The goal is to find the sweet spot that maximizes the overlap between these two segments. To do this, we want to gather data for three segments:
This process is repeated for one, two, three, and so on messages sent. Here’s what you’ll see if you set this up in a table on a spreadsheet.
Here you can see that users who sent at least three messages on the day of signing up have the biggest overlap with users who continue using Whatsapp after 21 days.
That’s why Whatsapp’s PAI is: three messages sent on the day of signing up because they’re likely to continue using it going forward.
You can further visualize this using bar graphs. From this visual, it’s clear that this has the biggest overlap between the two segments.
4. Compare the retention curves and validate the PAI.
Next is to verify the PAI by comparing the retention curve of users who sent at least three messages on the day of signing up with the baseline retention.
The retention curve indicates that the retention rate of users who sent at least three messages on the day of signing up is almost double the retention rate of your baseline rate for all users after 21 days.
If you’re up for it and have the data chops, you can do a Logistic Regression. This is beyond the scope of this book and requires a level of understanding of correlation and regression analysis.
5. Communicate your PAI.
Finally, once you've explored the data, ran some regressions, and verified that your model works, you’re ready to explain it to others. The intention is to make it dead simple to talk about.
Communicate this to the marketing and sales teams to ensure they’re not just pursuing signups. They should also focus on reaching the Moment of Value Adoption and achieving the PAI. Also, ask the sales and customer support teams to offer a higher level of support and care for these users.
This is exactly what Wave, a company that provides financial services and software for small businesses, did. After identifying their PAI, the whole organization adopted it as a measure of user success. Other teams joined in, including the demand gen team, to focus on channels and acquisition strategies that increase signups to reach the PAI.
It’s important to note that PAI is just a term. Other companies may call it differently. Wave calls it the first activation event or FAE for short. Others call this “tier one” signups or leads. Whatever you end up naming it, make sure it’s communicated often and adopted by your entire organization.
Product Qualified Leads and the PAI
It’s worth touching on how the PAI relates to a product qualified lead (PQL). Are the PAI and the PQL the same metric?
Unlike Marketing Qualified Leads (MQLs), which base buying intent on arbitrary factors like email opens, whitepaper downloads, and webpage visits, PQLs are users who have achieved meaningful value in a product. It’s often used as a way to identify high-value users to help the sales and support teams discover which users they should focus their efforts on.
Whereas the PAI focuses on one key product action so that it’s easy to communicate within the organization, the PQL could involve multiple product engagement metrics. This includes but is not limited to the following:
To answer the question, yes, a PQL could be the same thing as a PAI, but it doesn’t have to be. It really depends on the organization and whether the resources are available to provide high-touch support to more leads. If the resources are there, consider lowering the requirements for a PQL and set it for the moment users achieve their desired outcome for the first time. (We’ll get into PQLs and the sales-assisted onboarding process in Chapter 11.)
Let’s circle back to Slack for a minute. By now, we know Slack’s PAI is when a team sends 2,000 messages. If there are only a handful of sales and support personnel at Slack, they could set the PQL equivalent to the PAI. But if they went on a hiring spree of sales and support folks, they could lower that bar and set it to the first time a team sends ten messages. At that level, there will be more PQLs the sales team can pursue.
Visually, if you look at a continuum of user engagement within your product, where one end is the moment users sign up, and the other end is the moment users achieve the PAI, the PQL could be anywhere in between.
Implementing a Product Analytics Tool
Everything we’ve talked about in this chapter assumes that you’re measuring and tracking key product engagement metrics. This means going beyond a basic Google Analytics (GA) implementation.
GA was built to analyze marketing spend and was never meant to accommodate the depth and sophistication of a modern customer journey. Unless you define and implement events ahead of time, you can’t measure product engagement. Even then, GA aggregates the data, so it’s not possible to determine the value specific users are receiving from a product.
To measure the success of your onboarding experience, it’s important to collect deeper insights and actionable information from your users. This can only come from detailed knowledge about how they interact with what you’ve built. When you have evidence regarding the specific actions of users and what they like best, you can engage them longer, further upsell them, and keep them happy so they come back again and again.
Whether it’s Mixpanel, Heap Analytics, Amplitude, Pendo, or another product analytics tool, it is important to track meaningful product events and metrics.
For example, the key product engagement metrics for a B2B productivity tool could be:
For a social networking application, key product engagement metrics could be connections made, published content, posts liked, and comments made.
The point is that your product is unique. Identify the key product engagement metrics that are important to your product. Then implement a product analytics tool to track those metrics for each individual user.
What’s Next
Once you’ve identified the PAI, the next step in the EUREKA framework is to evaluate your new users’ journey. We’re about to break down the user onboarding experience step-by-step to identify anything that could be limiting users from achieving your product’s PAI.
CHAPTER 7: Refine Your Onboarding Success Criteria
If you do not know where you are going, every road will get you nowhere.
- Henry A. Kissinger
Google Maps can be quite a marvel.
The vast majority of the time, it gets us where we need to go without having to think about it. We just plug in our destination, and boom, we’re there. So many of us have become so reliant on it that when it fails, everything goes awry.
I experienced this firsthand with my wife Joanna on a road trip from Toronto to Montreal. Unfamiliar with the streets of Montreal, I relied heavily on Google Maps to find our hotel. Low and behold, my phone lost signal after exiting an underground tunnel, and I received that unbearable error message, “GPS lost signal.”
We ended up driving over a bridge that led to the US-Canada border. That’s not so bad if we both had our Canadian passports, but we didn’t!
Immediately, we both started freaking out. How are we supposed to explain to the Canadian Border Agents that we’re not illegal immigrants trying to sneak into the United States of America, a.k.a. the land of the free?
Of course, we completely overreacted. The Canadian Border Services Agency officer we spoke with was understanding. After showing him our Ontario driver’s license and explaining the Google Maps failure, he laughed it off. All we had to do was exit on a ramp that led us back to where we needed to go.
This is a harmless story, where not knowing your destination can lead you down the wrong path. But it doesn’t always end well, especially with the cross-functional, highly-visible effort of improving user onboarding.
By now, you should have a clear picture of what your users’ desired outcomes are along with the functional, emotional, and social jobs they are hiring your product for. You should also now know the four progress-making forces that influence the decision to adopt or drop the product.
The next step is to define what success looks like for your user onboarding experience. There are two moments that matter the most to measure onboarding success:
These two success criteria are tied to key milestones in the user onboarding: the Moment of Value Experience and the Moment of Value Adoption.
Success Criteria #1: The First Strike
The first onboarding success criteria is helping users achieve their desired outcome, or Customer Job, as quickly as possible.
In Wes Bush’s Bowling Alley framework, this event is called the “First Strike.” In 10-pin bowling, this happens when all ten pins are knocked down with a bowling ball. (We’ll go in-depth into the Bowling Alley framework in the next chapter.)
In user onboarding, the First Strike is a necessary product action users must take to accomplish their desired outcome or Customer Job. Let me give some examples.
For some companies, this First Strike isn’t so obvious. Think about Facebook. What’s their First Strike? Is it a user liking, sharing, or commenting on posts?
Since those actions require users to have a few Facebook friends, the most important product action is to add a friend. That’s why Facebook prompts new users to connect with friends as quickly as possible. This happens early on in their onboarding process.
For “all-in-one” products that solve many problems, recognizing the First Strike is even more tricky. Imagine identifying the First Strike for a Swiss Army knife. Is it the knife, pen, screwdriver, compass, scissors, saw blade, fire starter, or laser pointer?
Many all-in-one B2B SaaS products can essentially be considered digital Swiss Army knives. How do you determine the First Strike in these cases?
This is where context and segment matter. Depending on the context of use and the segment, there could be different First Strikes. For example, users can accomplish a lot with Intercom:
So, what is Intercom’s First Strike?
It’s hard to decipher at first glance. But by segmenting Intercom’s users, you learn that users hire Intercom for three core Customer Jobs, all aligning with their three product lines:
As you can see, the First Strike is closely tied to your product’s Customer Job (or Jobs, for more complex products). It’s the first measure of success that new users are on the right track.
Success Criteria #2: The Product Adoption Indicator
The second measure of success is the moment users start using a product consistently.
One of the end goals of onboarding is helping users embrace new habits with a product. Habit-forming user onboarding requires users to experience the value of a product more than once. If new users have used the product enough times, they’re more likely to continue with it going forward.
For Slack, a team is not successfully onboarded until they’ve sent not one, not 10, but 2,000 messages. It’s at this threshold where they’ve found the teams who are likely to continue using it.
Since this onboarding success metric is a leading indicator of user retention and product adoption, I call this the Product Adoption Indicator, or PAI for short. It’s an early but strong signal that users are likely to continue using a product going forward. They have embraced the product and are very unlikely to return to their old habits.
This concept is not new—others call this the “magic number.” Here are some well-known examples:
Notice that the PAIs for each of these products are closely tied to retention.
Once users reach this point, you’ve set them up for success. They’re now ready to take the next step in the product’s customer journey. They’ve completed the initial loop of the user onboarding process: they’ve perceived, experienced, and adopted your product for the first time.
Good PAIs have a few common characteristics:
The Five Steps To Determine Your PAI
There are five steps to establishing a company’s PAI.
I’ll be using Whatsapp as an example for this exercise. All data used below are fictional and used for instructional purposes only.
1. Get a baseline measurement of your retention.
As previously discussed, one result from good onboarding is a lift in retention rate. Before making any changes to the user onboarding, you’ll want to first define what retention looks like.
One way to do this is to perform a cohort analysis using a retention chart and curve. This reflects the percentage of users who come back and perform any action with a product several days or weeks after signing up.
With analytics tools like Mixpanel or Amplitude, you should have readily available reports to visualize a product’s retention curve. You could also calculate this metric by manually using Excel or Google Sheets.
For example, let’s say 12,481 users downloaded your app on January 1, and you want to calculate the retention rate for the first seven days. You need to track:
Next, you need to divide the number of returning users by the total number of users, then multiply by 100.
Out of the 12,481 users who started on January 1, only 3,506 end up returning on January 2.
Day 1 retention is: (3,506/12,481)*100 = 28.1%.
Repeat the same process for the remaining days to calculate your retention table:
Plot this on a chart, with the days on the horizontal axis and the retention rates are on the vertical axis. By doing this, you can calculate the baseline retention curve for your current user onboarding experience.
2. Create a PAI hypothesis.
Once you’ve identified the baseline retention curve, it’s time to create a hypothesis about your retention indicator. It should look something like this:
If new users perform at least X number of [the one key product action] during the first Y days/weeks of signing up, they’re more likely to continue using our product after Z days/weeks.
To create your own, let’s back up to break it down:
The final hypothesis for this example is:
If new Whatsapp users send at least three messages on the day of signing up, they’re more likely to continue using it after 21 days.
3. Gather data to validate your hypothesis.
What we’re looking for here is to maximize the overlap between the following two segments:
Another way to visualize this is by using a Venn diagram. The idea is to maximize the surface area between the segment of users who continue using Whatsapp after 21 days and the segment of users who have sent at least X messages on the first day of signing up.
If you take a look at the segment of users who have sent at least nine messages on the day of signing up, many might continue to use Whatsapp after 21 days.
But there could be quite a few retained Whatsapp users who don’t meet that number. In this case, the threshold for the PAI is too high. This is because we want to identify the minimum number of messages that also correspond to users who continue to use Whatsapp after 21 days.
On the other end, most users who send at least one message on the day of signing up did not continue using Whatsapp after 21 days. In this case, the threshold is too low.
The goal is to find the sweet spot that maximizes the overlap between these two segments. To do this, we want to gather data for three segments:
This process is repeated for one, two, three, and so on messages sent. Here’s what you’ll see if you set this up in a table on a spreadsheet.
Here you can see that users who sent at least three messages on the day of signing up have the biggest overlap with users who continue using Whatsapp after 21 days.
That’s why Whatsapp’s PAI is: three messages sent on the day of signing up because they’re likely to continue using it going forward.
You can further visualize this using bar graphs. From this visual, it’s clear that this has the biggest overlap between the two segments.
4. Compare the retention curves and validate the PAI.
Next is to verify the PAI by comparing the retention curve of users who sent at least three messages on the day of signing up with the baseline retention.
The retention curve indicates that the retention rate of users who sent at least three messages on the day of signing up is almost double the retention rate of your baseline rate for all users after 21 days.
If you’re up for it and have the data chops, you can do a Logistic Regression. This is beyond the scope of this book and requires a level of understanding of correlation and regression analysis.
5. Communicate your PAI.
Finally, once you've explored the data, ran some regressions, and verified that your model works, you’re ready to explain it to others. The intention is to make it dead simple to talk about.
Communicate this to the marketing and sales teams to ensure they’re not just pursuing signups. They should also focus on reaching the Moment of Value Adoption and achieving the PAI. Also, ask the sales and customer support teams to offer a higher level of support and care for these users.
This is exactly what Wave, a company that provides financial services and software for small businesses, did. After identifying their PAI, the whole organization adopted it as a measure of user success. Other teams joined in, including the demand gen team, to focus on channels and acquisition strategies that increase signups to reach the PAI.
It’s important to note that PAI is just a term. Other companies may call it differently. Wave calls it the first activation event or FAE for short. Others call this “tier one” signups or leads. Whatever you end up naming it, make sure it’s communicated often and adopted by your entire organization.
Product Qualified Leads and the PAI
It’s worth touching on how the PAI relates to a product qualified lead (PQL). Are the PAI and the PQL the same metric?
Unlike Marketing Qualified Leads (MQLs), which base buying intent on arbitrary factors like email opens, whitepaper downloads, and webpage visits, PQLs are users who have achieved meaningful value in a product. It’s often used as a way to identify high-value users to help the sales and support teams discover which users they should focus their efforts on.
Whereas the PAI focuses on one key product action so that it’s easy to communicate within the organization, the PQL could involve multiple product engagement metrics. This includes but is not limited to the following:
To answer the question, yes, a PQL could be the same thing as a PAI, but it doesn’t have to be. It really depends on the organization and whether the resources are available to provide high-touch support to more leads. If the resources are there, consider lowering the requirements for a PQL and set it for the moment users achieve their desired outcome for the first time. (We’ll get into PQLs and the sales-assisted onboarding process in Chapter 11.)
Let’s circle back to Slack for a minute. By now, we know Slack’s PAI is when a team sends 2,000 messages. If there are only a handful of sales and support personnel at Slack, they could set the PQL equivalent to the PAI. But if they went on a hiring spree of sales and support folks, they could lower that bar and set it to the first time a team sends ten messages. At that level, there will be more PQLs the sales team can pursue.
Visually, if you look at a continuum of user engagement within your product, where one end is the moment users sign up, and the other end is the moment users achieve the PAI, the PQL could be anywhere in between.
Implementing a Product Analytics Tool
Everything we’ve talked about in this chapter assumes that you’re measuring and tracking key product engagement metrics. This means going beyond a basic Google Analytics (GA) implementation.
GA was built to analyze marketing spend and was never meant to accommodate the depth and sophistication of a modern customer journey. Unless you define and implement events ahead of time, you can’t measure product engagement. Even then, GA aggregates the data, so it’s not possible to determine the value specific users are receiving from a product.
To measure the success of your onboarding experience, it’s important to collect deeper insights and actionable information from your users. This can only come from detailed knowledge about how they interact with what you’ve built. When you have evidence regarding the specific actions of users and what they like best, you can engage them longer, further upsell them, and keep them happy so they come back again and again.
Whether it’s Mixpanel, Heap Analytics, Amplitude, Pendo, or another product analytics tool, it is important to track meaningful product events and metrics.
For example, the key product engagement metrics for a B2B productivity tool could be:
For a social networking application, key product engagement metrics could be connections made, published content, posts liked, and comments made.
The point is that your product is unique. Identify the key product engagement metrics that are important to your product. Then implement a product analytics tool to track those metrics for each individual user.
What’s Next
Once you’ve identified the PAI, the next step in the EUREKA framework is to evaluate your new users’ journey. We’re about to break down the user onboarding experience step-by-step to identify anything that could be limiting users from achieving your product’s PAI.