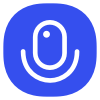
Sign up to save your podcasts
Or
This research paper explores the use of sparse autoencoders to extract interpretable features from Anthropic's Claude 3 Sonnet language model. The authors successfully scale this method to a large model, uncovering a diverse range of abstract features, including those related to safety concerns like bias, deception, and dangerous content. They investigate feature interpretability through examples and experiments, demonstrating that these features not only reflect but also causally influence model behavior. The study also examines the relationship between feature frequency and dictionary size, and compares the interpretability of features to that of individual neurons. Finally, the paper discusses the implications of these findings for AI safety and outlines future research directions.
Source: https://transformer-circuits.pub/2024/scaling-monosemanticity/
This research paper explores the use of sparse autoencoders to extract interpretable features from Anthropic's Claude 3 Sonnet language model. The authors successfully scale this method to a large model, uncovering a diverse range of abstract features, including those related to safety concerns like bias, deception, and dangerous content. They investigate feature interpretability through examples and experiments, demonstrating that these features not only reflect but also causally influence model behavior. The study also examines the relationship between feature frequency and dictionary size, and compares the interpretability of features to that of individual neurons. Finally, the paper discusses the implications of these findings for AI safety and outlines future research directions.
Source: https://transformer-circuits.pub/2024/scaling-monosemanticity/