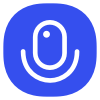
Sign up to save your podcasts
Or
Hey everyone, Ernis here, and welcome back to PaperLedge! Today, we're diving into a fascinating paper that tackles a HUGE problem in the world of AI: how do we actually know if these fancy Large Language Models – LLMs for short – are any good, especially when it comes to languages other than English?
Think of it like this: Imagine you're teaching a parrot to speak. You can easily test its English vocabulary, right? But what if you wanted it to speak Spanish, Portuguese, or even Basque? Suddenly, finding reliable ways to test its understanding becomes a lot harder. That's the challenge these researchers are addressing.
Most of the tests and leaderboards we use to rank LLMs are heavily focused on English. It's like judging the parrot's overall intelligence solely on its English skills. These tests also tend to focus on basic language skills, rather than the kinds of tasks businesses and organizations actually need these models to perform. And, once a test is created, it usually stays the same for a long time.
That's where IberBench comes in. These researchers created a brand-new benchmark – essentially a comprehensive test – specifically designed to evaluate LLMs on languages spoken across the Iberian Peninsula (think Spain and Portugal) and Ibero-America.
Now, what makes IberBench so special? Well, a few things:
The researchers then put 23 different LLMs, ranging in size from tiny to pretty darn big, through the IberBench wringer. And the results? Some interesting insights:
"LLMs perform worse on industry-relevant tasks than in fundamental ones."
This means that even if an LLM is great at basic language skills, it might struggle with tasks that are actually useful for businesses. It also turns out that some languages, like Galician and Basque, consistently see lower performance. And, shockingly, some tasks were basically a coin flip for the LLMs – they performed no better than random chance! For other tasks, LLMs did okay, but they still weren't as good as systems specifically designed for those tasks.
So, why does this matter? Well, for a few reasons:
And the best part? The entire evaluation pipeline, from the datasets to the leaderboard, is open-source. That means anyone can use it, contribute to it, and help make it even better!
So, what do you think, learning crew? Here are a couple of questions that popped into my head:
I'm excited to hear your thoughts on this one. Until next time, keep learning!
Hey everyone, Ernis here, and welcome back to PaperLedge! Today, we're diving into a fascinating paper that tackles a HUGE problem in the world of AI: how do we actually know if these fancy Large Language Models – LLMs for short – are any good, especially when it comes to languages other than English?
Think of it like this: Imagine you're teaching a parrot to speak. You can easily test its English vocabulary, right? But what if you wanted it to speak Spanish, Portuguese, or even Basque? Suddenly, finding reliable ways to test its understanding becomes a lot harder. That's the challenge these researchers are addressing.
Most of the tests and leaderboards we use to rank LLMs are heavily focused on English. It's like judging the parrot's overall intelligence solely on its English skills. These tests also tend to focus on basic language skills, rather than the kinds of tasks businesses and organizations actually need these models to perform. And, once a test is created, it usually stays the same for a long time.
That's where IberBench comes in. These researchers created a brand-new benchmark – essentially a comprehensive test – specifically designed to evaluate LLMs on languages spoken across the Iberian Peninsula (think Spain and Portugal) and Ibero-America.
Now, what makes IberBench so special? Well, a few things:
The researchers then put 23 different LLMs, ranging in size from tiny to pretty darn big, through the IberBench wringer. And the results? Some interesting insights:
"LLMs perform worse on industry-relevant tasks than in fundamental ones."
This means that even if an LLM is great at basic language skills, it might struggle with tasks that are actually useful for businesses. It also turns out that some languages, like Galician and Basque, consistently see lower performance. And, shockingly, some tasks were basically a coin flip for the LLMs – they performed no better than random chance! For other tasks, LLMs did okay, but they still weren't as good as systems specifically designed for those tasks.
So, why does this matter? Well, for a few reasons:
And the best part? The entire evaluation pipeline, from the datasets to the leaderboard, is open-source. That means anyone can use it, contribute to it, and help make it even better!
So, what do you think, learning crew? Here are a couple of questions that popped into my head:
I'm excited to hear your thoughts on this one. Until next time, keep learning!