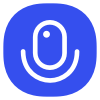
Sign up to save your podcasts
Or
Hey PaperLedge listeners, Ernis here, ready to dive into some seriously cool research! Today, we're talking about a new AI system that's tackling a really tricky problem: optimization.
Now, optimization might sound super technical, but you're doing it all the time! Imagine you're planning a road trip. You want to find the best route, balancing things like distance, gas costs, and maybe even scenic views. That's optimization in action!
But for scientists and engineers, optimization problems can get incredibly complex. They might involve designing the most efficient airplane wing, or figuring out how to allocate resources in a supply chain. The hard part is turning a real-world problem into a precise mathematical equation that a computer can solve. That's where OptimAI comes in.
This research introduces OptimAI, a new system that uses the power of Large Language Models (LLMs) – think of them as super-smart AI text generators – to automatically solve optimization problems described in plain English. The researchers found that OptimAI beats existing methods by a significant margin.
Here's how it works, and it's pretty clever:
First, there's the Formulator. This AI agent takes the natural language description of the problem and translates it into a formal mathematical equation. Think of it as a translator between human language and math language.
Next, we have the Planner. This agent comes up with a high-level strategy for solving the problem before any calculations are done. It's like drawing up a blueprint before starting construction.
Then come the Coder and Code Critic. The Coder writes the actual computer code to solve the problem, while the Code Critic checks the code for errors and suggests improvements. They work together like a coding team, constantly refining the solution.
The researchers found that all four of these roles are crucial. If you take away the Planner or the Code Critic, the system's effectiveness drops dramatically. It's like trying to build a house without an architect or a quality inspector!
Here's a fun analogy: Imagine OptimAI is a team of chefs trying to create the perfect dish. The Formulator figures out what ingredients are available, the Planner decides on the overall recipe, the Coder actually cooks the dish, and the Code Critic tastes it and suggests improvements.
What's really interesting is that OptimAI uses something called UCB-based debug scheduling. This is a fancy way of saying that the system can dynamically switch between different solution plans if it detects a problem. It's like having a backup recipe in case the first one doesn't work out!
The research emphasizes the importance of teamwork. By combining different AI models within one system, OptimAI achieves impressive results. In fact, it achieved significantly higher accuracy on benchmark datasets compared to previous methods.
So, why does this research matter? Well, for scientists and engineers, OptimAI could save them a huge amount of time and effort in formulating and solving optimization problems. It could also lead to better solutions in areas like logistics, manufacturing, and even drug discovery.
But even if you're not a scientist, this research shows the potential of AI to tackle complex real-world problems. It highlights the power of collaboration, both between humans and machines, and between different AI agents.
Here are a few questions that popped into my head:
Could OptimAI eventually be used to solve everyday optimization problems, like planning the most efficient grocery shopping route or managing personal finances?
How can we ensure that AI systems like OptimAI are used ethically and responsibly, especially when they're applied to complex and sensitive problems?
What are the limits of this approach? Are there certain types of optimization problems that OptimAI just can't handle?
That's all for today's episode, folks! I hope you found this deep dive into OptimAI as fascinating as I did. Until next time, keep exploring!
Hey PaperLedge listeners, Ernis here, ready to dive into some seriously cool research! Today, we're talking about a new AI system that's tackling a really tricky problem: optimization.
Now, optimization might sound super technical, but you're doing it all the time! Imagine you're planning a road trip. You want to find the best route, balancing things like distance, gas costs, and maybe even scenic views. That's optimization in action!
But for scientists and engineers, optimization problems can get incredibly complex. They might involve designing the most efficient airplane wing, or figuring out how to allocate resources in a supply chain. The hard part is turning a real-world problem into a precise mathematical equation that a computer can solve. That's where OptimAI comes in.
This research introduces OptimAI, a new system that uses the power of Large Language Models (LLMs) – think of them as super-smart AI text generators – to automatically solve optimization problems described in plain English. The researchers found that OptimAI beats existing methods by a significant margin.
Here's how it works, and it's pretty clever:
First, there's the Formulator. This AI agent takes the natural language description of the problem and translates it into a formal mathematical equation. Think of it as a translator between human language and math language.
Next, we have the Planner. This agent comes up with a high-level strategy for solving the problem before any calculations are done. It's like drawing up a blueprint before starting construction.
Then come the Coder and Code Critic. The Coder writes the actual computer code to solve the problem, while the Code Critic checks the code for errors and suggests improvements. They work together like a coding team, constantly refining the solution.
The researchers found that all four of these roles are crucial. If you take away the Planner or the Code Critic, the system's effectiveness drops dramatically. It's like trying to build a house without an architect or a quality inspector!
Here's a fun analogy: Imagine OptimAI is a team of chefs trying to create the perfect dish. The Formulator figures out what ingredients are available, the Planner decides on the overall recipe, the Coder actually cooks the dish, and the Code Critic tastes it and suggests improvements.
What's really interesting is that OptimAI uses something called UCB-based debug scheduling. This is a fancy way of saying that the system can dynamically switch between different solution plans if it detects a problem. It's like having a backup recipe in case the first one doesn't work out!
The research emphasizes the importance of teamwork. By combining different AI models within one system, OptimAI achieves impressive results. In fact, it achieved significantly higher accuracy on benchmark datasets compared to previous methods.
So, why does this research matter? Well, for scientists and engineers, OptimAI could save them a huge amount of time and effort in formulating and solving optimization problems. It could also lead to better solutions in areas like logistics, manufacturing, and even drug discovery.
But even if you're not a scientist, this research shows the potential of AI to tackle complex real-world problems. It highlights the power of collaboration, both between humans and machines, and between different AI agents.
Here are a few questions that popped into my head:
Could OptimAI eventually be used to solve everyday optimization problems, like planning the most efficient grocery shopping route or managing personal finances?
How can we ensure that AI systems like OptimAI are used ethically and responsibly, especially when they're applied to complex and sensitive problems?
What are the limits of this approach? Are there certain types of optimization problems that OptimAI just can't handle?
That's all for today's episode, folks! I hope you found this deep dive into OptimAI as fascinating as I did. Until next time, keep exploring!