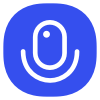
Sign up to save your podcasts
Or
Hey PaperLedge crew, Ernis here, ready to dive into another fascinating piece of research! This time, we're tackling a topic that's becoming increasingly important as AI gets smarter and more integrated into our lives: the safety of Large Reasoning Models, or LRMs. Think of LRMs as the super-smart cousins of the AI models that power things like chatbots and translation apps. They're really good at things that require complex thinking, like solving math problems or writing code.
Now, imagine giving someone incredibly powerful tools, like a super-fast car or a laser beam. You'd want to make sure they know how to use them safely, right? Well, that's the challenge we face with LRMs. As they get better at reasoning, they also become potentially vulnerable to misuse or unintended consequences. That's where this paper comes in.
Basically, the researchers have created a comprehensive map of the potential dangers lurking within these advanced AI systems. They've identified and categorized the different ways LRMs can be attacked, exploited, or used in ways that could cause harm. It’s like creating a safety manual for these super-smart AI systems. They cover a wide range of things, including:
The paper organizes all this information into a structured framework, a taxonomy, which is just a fancy word for a way of classifying things. This makes it easier for researchers and developers to understand the current safety landscape and develop better ways to secure these powerful models. It's like having a detailed blueprint of the vulnerabilities, allowing us to build stronger defenses.
Why does this matter? Well, for:
This research is crucial because LRMs are already being used in various applications, from medical diagnosis to financial analysis. If these systems are vulnerable, it could have serious consequences. Imagine an LRM used in self-driving cars being tricked into making a wrong turn, or an LRM used in fraud detection being manipulated to overlook suspicious transactions. That's the kind of scenario we want to prevent.
To really illustrate the importance, think about this: If we're going to trust AI with important decisions, we need to be absolutely sure that it's making those decisions based on accurate information and sound reasoning, not because it's been tricked or manipulated. This paper helps us get closer to that goal.
So, as we wrap up this preview, here are a couple of questions that might pop up during our full discussion:
I'm really excited to delve deeper into this topic with you all. Join me next time on PaperLedge as we explore the fascinating, and sometimes unsettling, world of Large Reasoning Model safety. Until then, keep learning, stay curious, and as always, thanks for listening!
Hey PaperLedge crew, Ernis here, ready to dive into another fascinating piece of research! This time, we're tackling a topic that's becoming increasingly important as AI gets smarter and more integrated into our lives: the safety of Large Reasoning Models, or LRMs. Think of LRMs as the super-smart cousins of the AI models that power things like chatbots and translation apps. They're really good at things that require complex thinking, like solving math problems or writing code.
Now, imagine giving someone incredibly powerful tools, like a super-fast car or a laser beam. You'd want to make sure they know how to use them safely, right? Well, that's the challenge we face with LRMs. As they get better at reasoning, they also become potentially vulnerable to misuse or unintended consequences. That's where this paper comes in.
Basically, the researchers have created a comprehensive map of the potential dangers lurking within these advanced AI systems. They've identified and categorized the different ways LRMs can be attacked, exploited, or used in ways that could cause harm. It’s like creating a safety manual for these super-smart AI systems. They cover a wide range of things, including:
The paper organizes all this information into a structured framework, a taxonomy, which is just a fancy word for a way of classifying things. This makes it easier for researchers and developers to understand the current safety landscape and develop better ways to secure these powerful models. It's like having a detailed blueprint of the vulnerabilities, allowing us to build stronger defenses.
Why does this matter? Well, for:
This research is crucial because LRMs are already being used in various applications, from medical diagnosis to financial analysis. If these systems are vulnerable, it could have serious consequences. Imagine an LRM used in self-driving cars being tricked into making a wrong turn, or an LRM used in fraud detection being manipulated to overlook suspicious transactions. That's the kind of scenario we want to prevent.
To really illustrate the importance, think about this: If we're going to trust AI with important decisions, we need to be absolutely sure that it's making those decisions based on accurate information and sound reasoning, not because it's been tricked or manipulated. This paper helps us get closer to that goal.
So, as we wrap up this preview, here are a couple of questions that might pop up during our full discussion:
I'm really excited to delve deeper into this topic with you all. Join me next time on PaperLedge as we explore the fascinating, and sometimes unsettling, world of Large Reasoning Model safety. Until then, keep learning, stay curious, and as always, thanks for listening!