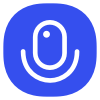
Sign up to save your podcasts
Or
Alright learning crew, Ernis here, ready to dive into some fascinating research! Today, we're tackling a paper about how we can trust the answers we get from those super-smart AI language models, like the ones that write emails for us or answer our burning questions online.
Think of it this way: Imagine you're writing a research paper, but instead of hitting the library, you have a super-powered AI assistant. This assistant uses something called Retrieval-Augmented Generation, or RAG for short. Basically, RAG lets the AI look up information in a bunch of documents – like a digital library – and then use that information to answer your questions, with citations, just like a real research paper!
Now, here's the kicker: how do we know if the AI is actually telling the truth, or if it's just making things up? This is what researchers call hallucination, and it's a big problem. We want to make sure that the information in those citations actually supports the AI's answer.
This paper dives deep into how we can evaluate whether the AI's answer is backed up by solid evidence. They looked at something called the TREC 2024 RAG Track, which is like a big competition where different teams submit their RAG systems. The researchers compared how well an AI judge (GPT-4o, a really powerful version of GPT) agreed with human judges on whether the AI's answers were supported by the cited documents.
Imagine it like this: you have a statement, say "Dogs make great pets because they are loyal." Now you have a source document that says "Dogs are known for their unwavering loyalty to their owners." Does the source document support the statement? That's the sort of thing these judges, both human and AI, are trying to determine.
They did this in two ways:
So, what did they find? Well, in over half the cases (56%), the AI judge (GPT-4o) and the human judges agreed perfectly from the start! And when the human judges could edit the AI's predictions, they agreed even more often (72%). That's pretty impressive!
But here's the really interesting part. The researchers found that when the human and AI judges disagreed, another independent human judge actually agreed more often with the AI judge than with the original human judge! This suggests that the AI judge might actually be pretty good at this, maybe even as good as, or in some cases better than, human judges at determining support.
The researchers concluded that "LLM judges can be a reliable alternative for support assessment."
Why does this matter?
This research is a step towards making AI more reliable and transparent. By understanding how well AI can assess its own answers, we can build systems that are less prone to errors and more helpful to everyone.
So, what does this all mean for the future of AI? Here are a couple of questions that popped into my head:
That's all for today's deep dive, learning crew! Stay curious, and keep questioning!
Alright learning crew, Ernis here, ready to dive into some fascinating research! Today, we're tackling a paper about how we can trust the answers we get from those super-smart AI language models, like the ones that write emails for us or answer our burning questions online.
Think of it this way: Imagine you're writing a research paper, but instead of hitting the library, you have a super-powered AI assistant. This assistant uses something called Retrieval-Augmented Generation, or RAG for short. Basically, RAG lets the AI look up information in a bunch of documents – like a digital library – and then use that information to answer your questions, with citations, just like a real research paper!
Now, here's the kicker: how do we know if the AI is actually telling the truth, or if it's just making things up? This is what researchers call hallucination, and it's a big problem. We want to make sure that the information in those citations actually supports the AI's answer.
This paper dives deep into how we can evaluate whether the AI's answer is backed up by solid evidence. They looked at something called the TREC 2024 RAG Track, which is like a big competition where different teams submit their RAG systems. The researchers compared how well an AI judge (GPT-4o, a really powerful version of GPT) agreed with human judges on whether the AI's answers were supported by the cited documents.
Imagine it like this: you have a statement, say "Dogs make great pets because they are loyal." Now you have a source document that says "Dogs are known for their unwavering loyalty to their owners." Does the source document support the statement? That's the sort of thing these judges, both human and AI, are trying to determine.
They did this in two ways:
So, what did they find? Well, in over half the cases (56%), the AI judge (GPT-4o) and the human judges agreed perfectly from the start! And when the human judges could edit the AI's predictions, they agreed even more often (72%). That's pretty impressive!
But here's the really interesting part. The researchers found that when the human and AI judges disagreed, another independent human judge actually agreed more often with the AI judge than with the original human judge! This suggests that the AI judge might actually be pretty good at this, maybe even as good as, or in some cases better than, human judges at determining support.
The researchers concluded that "LLM judges can be a reliable alternative for support assessment."
Why does this matter?
This research is a step towards making AI more reliable and transparent. By understanding how well AI can assess its own answers, we can build systems that are less prone to errors and more helpful to everyone.
So, what does this all mean for the future of AI? Here are a couple of questions that popped into my head:
That's all for today's deep dive, learning crew! Stay curious, and keep questioning!