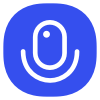
Sign up to save your podcasts
Or
Hey PaperLedge crew, Ernis here, ready to dive into some fascinating research!
Today, we're tackling a paper that looks at how to make those mega-powerful AI models, the ones that can write stories, answer questions, and even generate code, handle really, really long pieces of text. Think of it like this: a regular AI model has a hard time remembering the beginning of a novel by the time it gets to the end. These researchers are trying to give it a better memory!
The key idea is something called sparse attention. Now, "attention" in AI terms basically means "paying attention to" the important parts of the input. Regular attention is like trying to listen to everyone in a crowded room at once. Sparse attention, on the other hand, is like focusing on just a few key people you need to hear. This saves a ton of computational power.
Think of it like this: imagine you're trying to summarize a really long meeting. Do you need to remember every single word said? No! You focus on the key decisions, the main arguments, and the action items. Sparse attention does the same thing for AI.
So, what did these researchers actually do? They put different "sparse attention" methods to the test on a bunch of long-sequence tasks. They tinkered with the model size, how much "sparseness" to use, and even the length of the text the model was processing. They even created some new tasks specifically designed to be easy to evaluate – kind of like setting up a controlled science experiment.
Here are some of their key findings, translated into plain English:
So, why does all this matter? Well, for AI researchers, it gives them a better understanding of how to build these long-context AI models more efficiently. For businesses, it could lead to AI systems that can process massive amounts of data, like analyzing years of customer feedback or summarizing entire legal documents. For the average person, it could mean better AI assistants that can actually remember what you told them earlier in the conversation!
But it also highlights the importance of careful evaluation. Just because a technique sounds good in theory doesn't mean it'll work perfectly in practice.
Here are a couple of questions that popped into my head:
That's all for this episode! Let me know what you think of sparse attention and if you think it's the key to unlock better AI. Until next time, keep learning!
Hey PaperLedge crew, Ernis here, ready to dive into some fascinating research!
Today, we're tackling a paper that looks at how to make those mega-powerful AI models, the ones that can write stories, answer questions, and even generate code, handle really, really long pieces of text. Think of it like this: a regular AI model has a hard time remembering the beginning of a novel by the time it gets to the end. These researchers are trying to give it a better memory!
The key idea is something called sparse attention. Now, "attention" in AI terms basically means "paying attention to" the important parts of the input. Regular attention is like trying to listen to everyone in a crowded room at once. Sparse attention, on the other hand, is like focusing on just a few key people you need to hear. This saves a ton of computational power.
Think of it like this: imagine you're trying to summarize a really long meeting. Do you need to remember every single word said? No! You focus on the key decisions, the main arguments, and the action items. Sparse attention does the same thing for AI.
So, what did these researchers actually do? They put different "sparse attention" methods to the test on a bunch of long-sequence tasks. They tinkered with the model size, how much "sparseness" to use, and even the length of the text the model was processing. They even created some new tasks specifically designed to be easy to evaluate – kind of like setting up a controlled science experiment.
Here are some of their key findings, translated into plain English:
So, why does all this matter? Well, for AI researchers, it gives them a better understanding of how to build these long-context AI models more efficiently. For businesses, it could lead to AI systems that can process massive amounts of data, like analyzing years of customer feedback or summarizing entire legal documents. For the average person, it could mean better AI assistants that can actually remember what you told them earlier in the conversation!
But it also highlights the importance of careful evaluation. Just because a technique sounds good in theory doesn't mean it'll work perfectly in practice.
Here are a couple of questions that popped into my head:
That's all for this episode! Let me know what you think of sparse attention and if you think it's the key to unlock better AI. Until next time, keep learning!