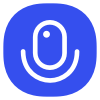
Sign up to save your podcasts
Or
The provided research paper explores the novel problem of continual forgetting in pre-trained vision models, where the goal is to sequentially remove specific unwanted knowledge while retaining the model's performance on other tasks. To address this, the authors introduce Group Sparse LoRA (GS-LoRA++), a parameter-efficient fine-tuning approach that uses low-rank adaptation with a group sparsity regularization to selectively modify the model's feed-forward networks. Furthermore, to handle practical scenarios like scarce forgetting data, they incorporate prototype regularization to guide the forgetting process. Extensive experiments across various vision tasks demonstrate the effectiveness and efficiency of their proposed method in achieving selective and continual knowledge erasure with minimal impact on remaining knowledge.
The provided research paper explores the novel problem of continual forgetting in pre-trained vision models, where the goal is to sequentially remove specific unwanted knowledge while retaining the model's performance on other tasks. To address this, the authors introduce Group Sparse LoRA (GS-LoRA++), a parameter-efficient fine-tuning approach that uses low-rank adaptation with a group sparsity regularization to selectively modify the model's feed-forward networks. Furthermore, to handle practical scenarios like scarce forgetting data, they incorporate prototype regularization to guide the forgetting process. Extensive experiments across various vision tasks demonstrate the effectiveness and efficiency of their proposed method in achieving selective and continual knowledge erasure with minimal impact on remaining knowledge.