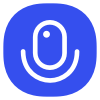
Sign up to save your podcasts
Or
Imagine a world where your smart glasses don't just identify objects but tell stories about what they see—all while running on a tiny battery without heating up. This cutting-edge vision is becoming reality as semiconductor companies tackle the monumental challenge of bringing generative AI capabilities from massive cloud data centers down to microcontroller-sized devices.
The semiconductor industry stands at a fascinating crossroads where artificial intelligence capabilities are pushing beyond traditional cloud environments into battery-powered edge devices. As our podcast guest explains, this transition faces substantial hurdles: while cloud-based models expand from millions to trillions of parameters, embedded systems must dramatically reduce their footprint from terabytes to gigabytes while still delivering meaningful AI functionality. With projections showing IoT devices consuming over 30 terabit hours of power by 2030 and generating 300 zettabytes of data, the need for local processing has never been more urgent.
For developers creating wearable technology like smart eyewear, constraints become particularly challenging. Weight distribution, battery life, and computing power must all be carefully balanced while maintaining comfort and style. The hardware architecture required for these applications demands innovative approaches: shared bus fabrics that enable different execution environments, strategic power management that activates high-performance cores only when needed, and neural processing units capable of handling transformer operations for generative AI workloads. Most impressively, current implementations demonstrate YOLO object detection running at just 60 milliamps—easily within battery operation parameters.
The $30 billion embedded AI market represents a tremendous opportunity for innovation, but also requires robust software ecosystems that help traditional microcontroller customers without AI expertise navigate this complex landscape. As next-generation devices begin supporting generative capabilities alongside traditional CNN and RNN networks, we're witnessing the dawn of truly seamless human-machine interfaces. Ready to explore how these technologies might transform your industry? Listen now to understand the future of computing at the edge.
Send us a text
Support the show
Learn more about the EDGE AI FOUNDATION - edgeaifoundation.org
Imagine a world where your smart glasses don't just identify objects but tell stories about what they see—all while running on a tiny battery without heating up. This cutting-edge vision is becoming reality as semiconductor companies tackle the monumental challenge of bringing generative AI capabilities from massive cloud data centers down to microcontroller-sized devices.
The semiconductor industry stands at a fascinating crossroads where artificial intelligence capabilities are pushing beyond traditional cloud environments into battery-powered edge devices. As our podcast guest explains, this transition faces substantial hurdles: while cloud-based models expand from millions to trillions of parameters, embedded systems must dramatically reduce their footprint from terabytes to gigabytes while still delivering meaningful AI functionality. With projections showing IoT devices consuming over 30 terabit hours of power by 2030 and generating 300 zettabytes of data, the need for local processing has never been more urgent.
For developers creating wearable technology like smart eyewear, constraints become particularly challenging. Weight distribution, battery life, and computing power must all be carefully balanced while maintaining comfort and style. The hardware architecture required for these applications demands innovative approaches: shared bus fabrics that enable different execution environments, strategic power management that activates high-performance cores only when needed, and neural processing units capable of handling transformer operations for generative AI workloads. Most impressively, current implementations demonstrate YOLO object detection running at just 60 milliamps—easily within battery operation parameters.
The $30 billion embedded AI market represents a tremendous opportunity for innovation, but also requires robust software ecosystems that help traditional microcontroller customers without AI expertise navigate this complex landscape. As next-generation devices begin supporting generative capabilities alongside traditional CNN and RNN networks, we're witnessing the dawn of truly seamless human-machine interfaces. Ready to explore how these technologies might transform your industry? Listen now to understand the future of computing at the edge.
Send us a text
Support the show
Learn more about the EDGE AI FOUNDATION - edgeaifoundation.org