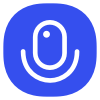
Sign up to save your podcasts
Or
In a video interview with The Forecast, DataRobot CEO Debanjan Saha discusses the need for finding value and building confidence to achieve enterprise AI success.
Find more enterprise cloud news, features stories and profiles at The Forecast.
Transcript (edited for readability):
Debanjan Seha: I was in a Morgan Stanley investment conference in San Francisco, and of course every investor quizzed me about ChatGPT and GeneAI. On the way back, the Uber driver quizzed me for half an hour about ChatGPT. And I realized that AI has arrived. But there are limitations to what some of these models can do. So what are those models doing? I mean, we have trained them on a really, really vast amount of publicly available data and sometimes on proprietary data. And once you run out of those data sources, there is a limit to how those models are going to get better and better over time. Of course, there will probably be better and better models, but I don’t think it can get incrementally better for an infinite amount of time. That’s not going to happen. Now, we are already pretty much fed all of these models with all the written text data that humankind has produced over the last hundreds of years.
So there is not too much text data left that you can feed these models to. What is left, by the way, is a lot of audio and video data, and that data is much easier to collect. And there are a lot of things which you don’t write down. For example, when you lift a cup from a table, nobody writes it down, but there are a lot of videos which show how we go and grab the cup and pick it up from the table. So those are the kind of data sources which are now going to make these models more and more intelligent. But I do think there is a limit to which you can feed them information and make them better.
[Related: Building a GenAI App to Improve Customer Support]
I see two gaps that we are really focused on. One I call the value gap. People are spending a lot of money training models, but ultimately those models have to solve some business problems. They have to make things more productive or predict things more accurately or whatever it is. Business value has to justify the investment that’s going in there. And it’s not easy with AI to create that business value. And one of the things DataRobot does very well is to connect business problems with AI to solve them. So that is the first problem that we are focused on. The second thing, which I also see with any new technology, is you need to build trust. AI is kind of in that phase and there is a confidence gap. There are a lot of people who are creating, especially with GenAI, a lot of prototypes.
[Related: Enterprise IT Teams Jump Into AIOps]
It’s not difficult to put together a chatbot which answers some questions. It’s hard to guarantee that they’re going to answer the question correctly, that it’s not going to create any misleading answers or create harm in more serious cases. And there are a whole lot of things we need to do in terms of managing the risk and creating a framework where you can define and associate risk with various different types of use cases and handle them with the right level of mitigation, compliance, testing and validation, et cetera. So those are the two things which are very, very important for wide scale adoption of AI.
[Related: Role of CIO Expands with Enterprise AI]
For example, the value gap. So the most interesting and most challenging thing about the value gap is that you need people who understand the business and the business challenges that they’re trying to solve. And you need people who understand AI and how those two things can be put together in order to solve a business problem. So we do ideation workshops with our customers. We sit down with customers and their people who understand the business and work with them to figure out their business challenges and what use cases they have. And then our team works with them to figure out how to address those use cases. Do they have all the ingredients…the right datasets? Do we know what business processes need to be updated if we insert AI into that? Those are the things we do first. Then people can use our platform either themselves or we can help them as co-pilots. And we sometimes have our other partners, the service provider partners, to work with them to solve those business problems and actually build those models, build those applications, and then put them in production.
Pretty much every industry you go to, there are enough problems that AI can help solve. And pretty much every business line you go to, there are enough problems that AI can help solve. But bringing AI to business, that’s still not easy for everybody because you need to speak both AI and the business language. And it’s very difficult to find people who speak both. So you have to have two in a box and solve those problems.
5
55 ratings
In a video interview with The Forecast, DataRobot CEO Debanjan Saha discusses the need for finding value and building confidence to achieve enterprise AI success.
Find more enterprise cloud news, features stories and profiles at The Forecast.
Transcript (edited for readability):
Debanjan Seha: I was in a Morgan Stanley investment conference in San Francisco, and of course every investor quizzed me about ChatGPT and GeneAI. On the way back, the Uber driver quizzed me for half an hour about ChatGPT. And I realized that AI has arrived. But there are limitations to what some of these models can do. So what are those models doing? I mean, we have trained them on a really, really vast amount of publicly available data and sometimes on proprietary data. And once you run out of those data sources, there is a limit to how those models are going to get better and better over time. Of course, there will probably be better and better models, but I don’t think it can get incrementally better for an infinite amount of time. That’s not going to happen. Now, we are already pretty much fed all of these models with all the written text data that humankind has produced over the last hundreds of years.
So there is not too much text data left that you can feed these models to. What is left, by the way, is a lot of audio and video data, and that data is much easier to collect. And there are a lot of things which you don’t write down. For example, when you lift a cup from a table, nobody writes it down, but there are a lot of videos which show how we go and grab the cup and pick it up from the table. So those are the kind of data sources which are now going to make these models more and more intelligent. But I do think there is a limit to which you can feed them information and make them better.
[Related: Building a GenAI App to Improve Customer Support]
I see two gaps that we are really focused on. One I call the value gap. People are spending a lot of money training models, but ultimately those models have to solve some business problems. They have to make things more productive or predict things more accurately or whatever it is. Business value has to justify the investment that’s going in there. And it’s not easy with AI to create that business value. And one of the things DataRobot does very well is to connect business problems with AI to solve them. So that is the first problem that we are focused on. The second thing, which I also see with any new technology, is you need to build trust. AI is kind of in that phase and there is a confidence gap. There are a lot of people who are creating, especially with GenAI, a lot of prototypes.
[Related: Enterprise IT Teams Jump Into AIOps]
It’s not difficult to put together a chatbot which answers some questions. It’s hard to guarantee that they’re going to answer the question correctly, that it’s not going to create any misleading answers or create harm in more serious cases. And there are a whole lot of things we need to do in terms of managing the risk and creating a framework where you can define and associate risk with various different types of use cases and handle them with the right level of mitigation, compliance, testing and validation, et cetera. So those are the two things which are very, very important for wide scale adoption of AI.
[Related: Role of CIO Expands with Enterprise AI]
For example, the value gap. So the most interesting and most challenging thing about the value gap is that you need people who understand the business and the business challenges that they’re trying to solve. And you need people who understand AI and how those two things can be put together in order to solve a business problem. So we do ideation workshops with our customers. We sit down with customers and their people who understand the business and work with them to figure out their business challenges and what use cases they have. And then our team works with them to figure out how to address those use cases. Do they have all the ingredients…the right datasets? Do we know what business processes need to be updated if we insert AI into that? Those are the things we do first. Then people can use our platform either themselves or we can help them as co-pilots. And we sometimes have our other partners, the service provider partners, to work with them to solve those business problems and actually build those models, build those applications, and then put them in production.
Pretty much every industry you go to, there are enough problems that AI can help solve. And pretty much every business line you go to, there are enough problems that AI can help solve. But bringing AI to business, that’s still not easy for everybody because you need to speak both AI and the business language. And it’s very difficult to find people who speak both. So you have to have two in a box and solve those problems.