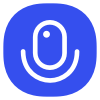
Sign up to save your podcasts
Or
This paper introduces a deep learning-based method for the generalized inverse design of complex multi-port radio-frequency and sub-terahertz electromagnetic structures and integrated circuits. This innovative approach uses deep neural networks to accurately predict the properties of arbitrary-shaped designs, significantly speeding up the synthesis process compared to traditional methods. The authors demonstrate the effectiveness of this technique through various examples, including antennas, filters, and even a broadband millimeter-wave amplifier, showcasing its potential to overcome limitations of conventional design approaches and explore a broader design space. The presented methodology enables a rapid and automated synthesis of sophisticated high-frequency circuits.
This paper introduces a deep learning-based method for the generalized inverse design of complex multi-port radio-frequency and sub-terahertz electromagnetic structures and integrated circuits. This innovative approach uses deep neural networks to accurately predict the properties of arbitrary-shaped designs, significantly speeding up the synthesis process compared to traditional methods. The authors demonstrate the effectiveness of this technique through various examples, including antennas, filters, and even a broadband millimeter-wave amplifier, showcasing its potential to overcome limitations of conventional design approaches and explore a broader design space. The presented methodology enables a rapid and automated synthesis of sophisticated high-frequency circuits.