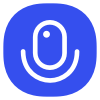
Sign up to save your podcasts
Or
Welcome to another episode of the AI Concepts Podcast, where we simplify complex AI topics into digestible explanations. This episode continues our Deep Learning series, diving into the limitations of Recurrent Neural Networks (RNNs) and introducing their game-changing successors: Long Short-Term Memory (LSTM) networks and Gated Recurrent Units (GRUs). Learn how these architectures revolutionize tasks with long-term dependencies by mastering memory control and selective information processing, paving the way for more advanced AI applications.
Explore the intricate workings of gates within LSTMs, which help in managing information flow for better memory retention, and delve into the lightweight efficiency of GRUs. Understand how these innovations bridge the gap between theoretical potential and practical efficiency in AI tasks like language processing and time series prediction.
Stay tuned for our next episode, where we’ll unravel the attention mechanism, a groundbreaking development that shifts the paradigm from memory reliance to direct input relevance, crucial for modern models like transformers.
Welcome to another episode of the AI Concepts Podcast, where we simplify complex AI topics into digestible explanations. This episode continues our Deep Learning series, diving into the limitations of Recurrent Neural Networks (RNNs) and introducing their game-changing successors: Long Short-Term Memory (LSTM) networks and Gated Recurrent Units (GRUs). Learn how these architectures revolutionize tasks with long-term dependencies by mastering memory control and selective information processing, paving the way for more advanced AI applications.
Explore the intricate workings of gates within LSTMs, which help in managing information flow for better memory retention, and delve into the lightweight efficiency of GRUs. Understand how these innovations bridge the gap between theoretical potential and practical efficiency in AI tasks like language processing and time series prediction.
Stay tuned for our next episode, where we’ll unravel the attention mechanism, a groundbreaking development that shifts the paradigm from memory reliance to direct input relevance, crucial for modern models like transformers.