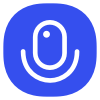
Sign up to save your podcasts
Or
This episode breaks down the 'Deep Residual Learning for Image Recognition' paper, which describes the development of a deep residual learning framework for image recognition. The authors address the "degradation problem" encountered when training very deep neural networks, where accuracy plateaus and degrades rapidly with increasing depth. They propose a novel approach that reformulates the layers to learn residual functions with reference to the layer inputs, making it easier to optimise and allowing for significant accuracy gains from increased depth. Their experiments on the ImageNet dataset with residual networks (ResNets) of up to 152 layers demonstrate a substantial improvement in accuracy compared to previous state-of-the-art models, leading to a 1st place win in the ILSVRC 2015 classification competition. The paper also investigates the effectiveness of ResNets in object detection and localisation tasks, achieving remarkable results on the PASCAL VOC and COCO datasets, further highlighting the generalisability and effectiveness of the residual learning principle.
Audio : (Spotify) https://open.spotify.com/episode/5CgOzdBnaLVtW8QcMURJId?si=fpNCTxNET86SodIpz0xhwQ
Paper: https://arxiv.org/abs/1512.03385
This episode breaks down the 'Deep Residual Learning for Image Recognition' paper, which describes the development of a deep residual learning framework for image recognition. The authors address the "degradation problem" encountered when training very deep neural networks, where accuracy plateaus and degrades rapidly with increasing depth. They propose a novel approach that reformulates the layers to learn residual functions with reference to the layer inputs, making it easier to optimise and allowing for significant accuracy gains from increased depth. Their experiments on the ImageNet dataset with residual networks (ResNets) of up to 152 layers demonstrate a substantial improvement in accuracy compared to previous state-of-the-art models, leading to a 1st place win in the ILSVRC 2015 classification competition. The paper also investigates the effectiveness of ResNets in object detection and localisation tasks, achieving remarkable results on the PASCAL VOC and COCO datasets, further highlighting the generalisability and effectiveness of the residual learning principle.
Audio : (Spotify) https://open.spotify.com/episode/5CgOzdBnaLVtW8QcMURJId?si=fpNCTxNET86SodIpz0xhwQ
Paper: https://arxiv.org/abs/1512.03385