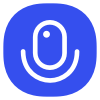
Sign up to save your podcasts
Or
DeepSeek-AI introduces DeepSeek-R1, a reasoning model developed through reinforcement learning (RL) and distillation techniques. The research explores two models: DeepSeek-R1-Zero, trained purely via RL, and DeepSeek-R1, which incorporates multi-stage training and "cold-start" data before RL to improve reasoning capabilities and readability. The paper highlights DeepSeek-R1-Zero's emergent reasoning behaviors and DeepSeek-R1's performance comparable to OpenAI's o1-1217 on reasoning tasks. Distillation from DeepSeek-R1 is used to create smaller, more efficient models, demonstrating that reasoning patterns can be effectively transferred. The research also details the challenges and unsuccessful attempts during development, such as using Process Reward Models and Monte Carlo Tree Search. The models and distilled versions are open-sourced to support further research in the community.
DeepSeek-AI introduces DeepSeek-R1, a reasoning model developed through reinforcement learning (RL) and distillation techniques. The research explores two models: DeepSeek-R1-Zero, trained purely via RL, and DeepSeek-R1, which incorporates multi-stage training and "cold-start" data before RL to improve reasoning capabilities and readability. The paper highlights DeepSeek-R1-Zero's emergent reasoning behaviors and DeepSeek-R1's performance comparable to OpenAI's o1-1217 on reasoning tasks. Distillation from DeepSeek-R1 is used to create smaller, more efficient models, demonstrating that reasoning patterns can be effectively transferred. The research also details the challenges and unsuccessful attempts during development, such as using Process Reward Models and Monte Carlo Tree Search. The models and distilled versions are open-sourced to support further research in the community.