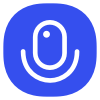
Sign up to save your podcasts
Or
DeepSeek-AI introduces DeepSeek-R1, a reasoning model developed through reinforcement learning (RL) and distillation techniques. The research explores how large language models can develop reasoning skills, even without supervised fine-tuning, highlighting the self-evolution observed in DeepSeek-R1-Zero during RL training. DeepSeek-R1 addresses limitations of DeepSeek-R1-Zero, like readability, by incorporating cold-start data and multi-stage training. Results demonstrate DeepSeek-R1 achieving performance comparable to OpenAI models on reasoning tasks, and distillation proves effective in empowering smaller models with enhanced reasoning capabilities. The study also shares unsuccessful attempts with Process Reward Models (PRM) and Monte Carlo Tree Search (MCTS), providing valuable insights into the challenges of improving reasoning in LLMs. The open-sourcing of the models aims to support further research in this area.
DeepSeek-AI introduces DeepSeek-R1, a reasoning model developed through reinforcement learning (RL) and distillation techniques. The research explores how large language models can develop reasoning skills, even without supervised fine-tuning, highlighting the self-evolution observed in DeepSeek-R1-Zero during RL training. DeepSeek-R1 addresses limitations of DeepSeek-R1-Zero, like readability, by incorporating cold-start data and multi-stage training. Results demonstrate DeepSeek-R1 achieving performance comparable to OpenAI models on reasoning tasks, and distillation proves effective in empowering smaller models with enhanced reasoning capabilities. The study also shares unsuccessful attempts with Process Reward Models (PRM) and Monte Carlo Tree Search (MCTS), providing valuable insights into the challenges of improving reasoning in LLMs. The open-sourcing of the models aims to support further research in this area.