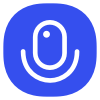
Sign up to save your podcasts
Or
This episode proposes a novel framework for evaluating large language models (LLMs) that prioritizes efficiency over sheer scale. Instead of focusing solely on model size and training data, it introduces the concept of "density," which measures performance relative to the number of parameters.
This allows for more equitable comparisons between models of varying sizes and reveals that smaller models can sometimes be more efficient.
The framework also incorporates "relative density" to benchmark against existing models. Ultimately, this new metric promotes the development of more resource-conscious AI systems.
This episode proposes a novel framework for evaluating large language models (LLMs) that prioritizes efficiency over sheer scale. Instead of focusing solely on model size and training data, it introduces the concept of "density," which measures performance relative to the number of parameters.
This allows for more equitable comparisons between models of varying sizes and reveals that smaller models can sometimes be more efficient.
The framework also incorporates "relative density" to benchmark against existing models. Ultimately, this new metric promotes the development of more resource-conscious AI systems.