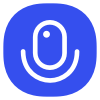
Sign up to save your podcasts
Or
Seventy3: 用NotebookLM将论文生成播客,让大家跟着AI一起进步。
今天的主题是:Large Concept Models: Language Modeling in a Sentence Representation SpaceSummary
This research paper introduces Large Concept Models (LCMs), a novel approach to language modeling that operates on sentence embeddings instead of individual tokens. LCMs aim to mimic human-like abstract reasoning by processing higher-level semantic representations, improving long-form text generation and zero-shot cross-lingual performance. The authors explore various LCM architectures, including those based on mean squared error regression and diffusion models, and evaluate their performance on summarization and a novel summary expansion task. Their findings demonstrate that diffusion-based LCMs outperform other methods, exhibiting impressive zero-shot generalization across multiple languages. The research also explores the concept of incorporating explicit planning into the model to further enhance coherence in long-form text generation.
本文提出了大型概念模型(LCMs),一种新颖的语言建模方法,其操作基于句子嵌入而非单独的词元。LCMs 旨在通过处理更高层次的语义表示来模拟类似人类的抽象推理,从而改进长篇文本生成和零样本跨语言性能。作者探讨了多种 LCM 架构,包括基于均方误差回归和扩散模型的架构,并在摘要生成和一种新颖的摘要扩展任务上评估了它们的性能。研究结果表明,基于扩散的 LCMs 表现优于其他方法,在多种语言上的零样本泛化能力令人印象深刻。研究还探讨了在模型中引入显式规划的概念,以进一步增强长篇文本生成的连贯性。
原文链接:https://arxiv.org/abs/2412.08821
Seventy3: 用NotebookLM将论文生成播客,让大家跟着AI一起进步。
今天的主题是:Large Concept Models: Language Modeling in a Sentence Representation SpaceSummary
This research paper introduces Large Concept Models (LCMs), a novel approach to language modeling that operates on sentence embeddings instead of individual tokens. LCMs aim to mimic human-like abstract reasoning by processing higher-level semantic representations, improving long-form text generation and zero-shot cross-lingual performance. The authors explore various LCM architectures, including those based on mean squared error regression and diffusion models, and evaluate their performance on summarization and a novel summary expansion task. Their findings demonstrate that diffusion-based LCMs outperform other methods, exhibiting impressive zero-shot generalization across multiple languages. The research also explores the concept of incorporating explicit planning into the model to further enhance coherence in long-form text generation.
本文提出了大型概念模型(LCMs),一种新颖的语言建模方法,其操作基于句子嵌入而非单独的词元。LCMs 旨在通过处理更高层次的语义表示来模拟类似人类的抽象推理,从而改进长篇文本生成和零样本跨语言性能。作者探讨了多种 LCM 架构,包括基于均方误差回归和扩散模型的架构,并在摘要生成和一种新颖的摘要扩展任务上评估了它们的性能。研究结果表明,基于扩散的 LCMs 表现优于其他方法,在多种语言上的零样本泛化能力令人印象深刻。研究还探讨了在模型中引入显式规划的概念,以进一步增强长篇文本生成的连贯性。
原文链接:https://arxiv.org/abs/2412.08821