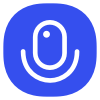
Sign up to save your podcasts
Or
Seventy3: 用NotebookLM将论文生成播客,让大家跟着AI一起进步。
今天的主题是:MaxInfoRL: Boosting exploration in reinforcement learning through information gain maximizationSummary
The paper introduces MAXINFORL, a novel reinforcement learning (RL) framework that improves exploration by maximizing information gain about the underlying task. It augments existing off-policy RL methods with directed exploration, using intrinsic rewards derived from model epistemic uncertainty to guide exploration more effectively than standard methods like ϵ-greedy or Boltzmann exploration. Theoretical analysis shows sublinear regret in a simplified multi-armed bandit setting, and empirical results demonstrate superior performance across various deep RL benchmarks, including challenging visual control tasks. The authors propose an auto-tuning procedure for balancing intrinsic and extrinsic exploration objectives, enhancing simplicity and scalability. Finally, the paper discusses related work and potential future research directions.
本文提出了MAXINFORL,一种新型的强化学习(RL)框架,通过最大化对底层任务的信息增益来改进探索能力。该框架将现有的离策略强化学习方法与定向探索相结合,利用源于模型认知不确定性的内在奖励来比标准方法(如 ϵ-greedy 或 Boltzmann 探索)更有效地引导探索。理论分析表明,在简化的多臂老虎机场景中具有次线性遗憾值,实验证明其在各种深度强化学习基准测试(包括具有挑战性的视觉控制任务)中的优越性能。作者提出了一种自动调节内在与外在探索目标平衡的程序,以提升方法的简洁性和可扩展性。最后,论文讨论了相关工作以及未来潜在的研究方向。
原文链接:https://arxiv.org/abs/2412.12098
Seventy3: 用NotebookLM将论文生成播客,让大家跟着AI一起进步。
今天的主题是:MaxInfoRL: Boosting exploration in reinforcement learning through information gain maximizationSummary
The paper introduces MAXINFORL, a novel reinforcement learning (RL) framework that improves exploration by maximizing information gain about the underlying task. It augments existing off-policy RL methods with directed exploration, using intrinsic rewards derived from model epistemic uncertainty to guide exploration more effectively than standard methods like ϵ-greedy or Boltzmann exploration. Theoretical analysis shows sublinear regret in a simplified multi-armed bandit setting, and empirical results demonstrate superior performance across various deep RL benchmarks, including challenging visual control tasks. The authors propose an auto-tuning procedure for balancing intrinsic and extrinsic exploration objectives, enhancing simplicity and scalability. Finally, the paper discusses related work and potential future research directions.
本文提出了MAXINFORL,一种新型的强化学习(RL)框架,通过最大化对底层任务的信息增益来改进探索能力。该框架将现有的离策略强化学习方法与定向探索相结合,利用源于模型认知不确定性的内在奖励来比标准方法(如 ϵ-greedy 或 Boltzmann 探索)更有效地引导探索。理论分析表明,在简化的多臂老虎机场景中具有次线性遗憾值,实验证明其在各种深度强化学习基准测试(包括具有挑战性的视觉控制任务)中的优越性能。作者提出了一种自动调节内在与外在探索目标平衡的程序,以提升方法的简洁性和可扩展性。最后,论文讨论了相关工作以及未来潜在的研究方向。
原文链接:https://arxiv.org/abs/2412.12098