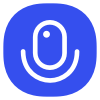
Sign up to save your podcasts
Or
Seventy3: 用NotebookLM将论文生成播客,让大家跟着AI一起进步。
今天的主题是:ScaleOT: Privacy-utility-scalable Offsite-tuning with Dynamic LayerReplace and Selective Rank CompressionSummary
This research introduces ScaleOT, a novel framework for privacy-preserving offsite tuning of large language models (LLMs). ScaleOT addresses limitations of existing methods by using reinforcement learning to determine layer importance, replacing less important layers with lightweight networks ("harmonizers"), and employing rank reduction to further compress the model. The resulting emulators balance privacy and utility, enabling effective downstream task tuning while protecting both model and data privacy. Extensive experiments demonstrate ScaleOT's superior performance compared to state-of-the-art methods across various LLMs and tasks. The approach is shown to be compatible with parameter-efficient fine-tuning techniques.
本文提出了ScaleOT,一种用于保护隐私的大型语言模型(LLMs)离站微调的新型框架。ScaleOT 通过强化学习确定模型层的重要性,将较不重要的层替换为轻量级网络(称为“协调器”),并采用秩减技术进一步压缩模型,从而克服了现有方法的局限性。生成的模拟器在隐私与实用性之间实现了平衡,能够在保护模型和数据隐私的同时,有效地进行下游任务微调。大量实验表明,ScaleOT 在多种 LLM 和任务上的性能优于当前最先进的方法。研究还表明,该方法兼容参数高效微调技术。
原文链接:https://arxiv.org/abs/2412.09812
Seventy3: 用NotebookLM将论文生成播客,让大家跟着AI一起进步。
今天的主题是:ScaleOT: Privacy-utility-scalable Offsite-tuning with Dynamic LayerReplace and Selective Rank CompressionSummary
This research introduces ScaleOT, a novel framework for privacy-preserving offsite tuning of large language models (LLMs). ScaleOT addresses limitations of existing methods by using reinforcement learning to determine layer importance, replacing less important layers with lightweight networks ("harmonizers"), and employing rank reduction to further compress the model. The resulting emulators balance privacy and utility, enabling effective downstream task tuning while protecting both model and data privacy. Extensive experiments demonstrate ScaleOT's superior performance compared to state-of-the-art methods across various LLMs and tasks. The approach is shown to be compatible with parameter-efficient fine-tuning techniques.
本文提出了ScaleOT,一种用于保护隐私的大型语言模型(LLMs)离站微调的新型框架。ScaleOT 通过强化学习确定模型层的重要性,将较不重要的层替换为轻量级网络(称为“协调器”),并采用秩减技术进一步压缩模型,从而克服了现有方法的局限性。生成的模拟器在隐私与实用性之间实现了平衡,能够在保护模型和数据隐私的同时,有效地进行下游任务微调。大量实验表明,ScaleOT 在多种 LLM 和任务上的性能优于当前最先进的方法。研究还表明,该方法兼容参数高效微调技术。
原文链接:https://arxiv.org/abs/2412.09812