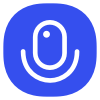
Sign up to save your podcasts
Or
Seventy3: 用NotebookLM将论文生成播客,让大家跟着AI一起进步。
今天的主题是:No More Adam: Learning Rate Scaling at Initialization is All You NeedSummary
The research introduces SGD-SaI, a novel optimization method that significantly improves the memory efficiency and training speed of large neural networks. Unlike adaptive methods like AdamW, SGD-SaI scales learning rates at initialization based on gradient signal-to-noise ratios, eliminating the need for storing and updating second-order momentum. This approach achieves performance comparable to or exceeding AdamW across various tasks, including large language model and vision transformer training. The study empirically validates SGD-SaI's effectiveness and efficiency, demonstrating its superior robustness to hyperparameter variations and scalability to large models. The authors conclude that SGD-SaI offers a simpler, more efficient alternative to adaptive gradient methods for training deep neural networks.
本研究提出了SGD-SaI,一种新型的优化方法,大幅提升了大型神经网络的内存效率和训练速度。与 AdamW 等自适应方法不同,SGD-SaI 基于梯度信噪比在初始化时动态调整学习率,从而无需存储和更新二阶动量。该方法在包括大型语言模型和视觉 Transformer 训练在内的多种任务中表现出与 AdamW 相当或更优的性能。研究通过实验验证了 SGD-SaI 的高效性和有效性,展现了其对超参数变化的更强鲁棒性以及对大模型的良好扩展性。作者总结道,SGD-SaI 为深度神经网络训练提供了一种更简单、高效的替代自适应梯度方法的解决方案。
原文链接:https://arxiv.org/abs/2412.11768
Seventy3: 用NotebookLM将论文生成播客,让大家跟着AI一起进步。
今天的主题是:No More Adam: Learning Rate Scaling at Initialization is All You NeedSummary
The research introduces SGD-SaI, a novel optimization method that significantly improves the memory efficiency and training speed of large neural networks. Unlike adaptive methods like AdamW, SGD-SaI scales learning rates at initialization based on gradient signal-to-noise ratios, eliminating the need for storing and updating second-order momentum. This approach achieves performance comparable to or exceeding AdamW across various tasks, including large language model and vision transformer training. The study empirically validates SGD-SaI's effectiveness and efficiency, demonstrating its superior robustness to hyperparameter variations and scalability to large models. The authors conclude that SGD-SaI offers a simpler, more efficient alternative to adaptive gradient methods for training deep neural networks.
本研究提出了SGD-SaI,一种新型的优化方法,大幅提升了大型神经网络的内存效率和训练速度。与 AdamW 等自适应方法不同,SGD-SaI 基于梯度信噪比在初始化时动态调整学习率,从而无需存储和更新二阶动量。该方法在包括大型语言模型和视觉 Transformer 训练在内的多种任务中表现出与 AdamW 相当或更优的性能。研究通过实验验证了 SGD-SaI 的高效性和有效性,展现了其对超参数变化的更强鲁棒性以及对大模型的良好扩展性。作者总结道,SGD-SaI 为深度神经网络训练提供了一种更简单、高效的替代自适应梯度方法的解决方案。
原文链接:https://arxiv.org/abs/2412.11768