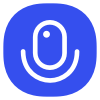
Sign up to save your podcasts
Or
Seventy3: 用NotebookLM将论文生成播客,让大家跟着AI一起进步。
今天的主题是:Deliberation in Latent Space via Differentiable Cache AugmentationSummary
This research paper explores a novel method for improving large language models (LLMs) by augmenting their internal cache with latent embeddings generated by a separate "coprocessor" model. This coprocessor, trained using standard language modeling techniques on a large dataset, learns to distill additional computation into the LLM's cache, enhancing its reasoning abilities without modifying the LLM's architecture. The approach allows for offline and asynchronous operation, improving efficiency and performance across a range of reasoning tasks. Experiments demonstrate consistent improvements in perplexity and accuracy on various benchmarks, showcasing the effectiveness of this differentiable cache augmentation technique. The method is compared to existing techniques, such as pause tokens and chain-of-thought prompting, showing superior performance.
本文探讨了一种新方法,通过在大型语言模型(LLMs)的内部缓存中增加由单独的“协处理器”模型生成的潜在嵌入,来提升 LLM 的性能。该协处理器使用标准语言建模技术在大型数据集上训练,学会将额外的计算提炼到 LLM 的缓存中,从而在不修改 LLM 架构的情况下增强其推理能力。该方法支持离线和异步操作,提高了多种推理任务的效率和性能。实验表明,在各种基准测试中,该方法在困惑度和准确性方面均实现了持续改进,展现了这种可微缓存增强技术的有效性。与现有技术(如暂停标记和链式思维提示)相比,该方法表现出更优越的性能。
原文链接:https://arxiv.org/abs/2412.17747
Seventy3: 用NotebookLM将论文生成播客,让大家跟着AI一起进步。
今天的主题是:Deliberation in Latent Space via Differentiable Cache AugmentationSummary
This research paper explores a novel method for improving large language models (LLMs) by augmenting their internal cache with latent embeddings generated by a separate "coprocessor" model. This coprocessor, trained using standard language modeling techniques on a large dataset, learns to distill additional computation into the LLM's cache, enhancing its reasoning abilities without modifying the LLM's architecture. The approach allows for offline and asynchronous operation, improving efficiency and performance across a range of reasoning tasks. Experiments demonstrate consistent improvements in perplexity and accuracy on various benchmarks, showcasing the effectiveness of this differentiable cache augmentation technique. The method is compared to existing techniques, such as pause tokens and chain-of-thought prompting, showing superior performance.
本文探讨了一种新方法,通过在大型语言模型(LLMs)的内部缓存中增加由单独的“协处理器”模型生成的潜在嵌入,来提升 LLM 的性能。该协处理器使用标准语言建模技术在大型数据集上训练,学会将额外的计算提炼到 LLM 的缓存中,从而在不修改 LLM 架构的情况下增强其推理能力。该方法支持离线和异步操作,提高了多种推理任务的效率和性能。实验表明,在各种基准测试中,该方法在困惑度和准确性方面均实现了持续改进,展现了这种可微缓存增强技术的有效性。与现有技术(如暂停标记和链式思维提示)相比,该方法表现出更优越的性能。
原文链接:https://arxiv.org/abs/2412.17747