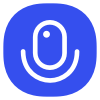
Sign up to save your podcasts
Or
Seventy3: 用NotebookLM将论文生成播客,让大家跟着AI一起进步。
今天的主题是:Automating the Search for Artificial Life with Foundation ModelsSummary
This research paper introduces Automated Search for Artificial Life (ASAL), a novel method using foundation models (FMs) to automate the discovery of interesting artificial life (ALife) simulations. ASAL employs FMs to evaluate simulations across diverse substrates (like Boids and Lenia), enabling three search strategies: supervised target searching, open-endedness searching, and illumination. The approach successfully discovers unseen lifeforms and quantifies previously qualitative ALife phenomena, accelerating research by automating a traditionally manual and time-consuming process. The authors demonstrate ASAL's effectiveness through experiments and discuss future applications, including expanding to video and 3D simulations. Finally, the paper explores the implications of using different FMs and substrates, highlighting the importance of selecting appropriate models for specific research goals.
本文提出了自动化人工生命搜索(Automated Search for Artificial Life,ASAL),一种利用基础模型(FMs)自动发现有趣人工生命(ALife)模拟的新方法。ASAL 利用基础模型在多样化基质(如 Boids 和 Lenia)上评估模拟,支持三种搜索策略:有监督目标搜索、开放性搜索和照明搜索。该方法成功发现了未曾见过的生命形式,并将以往定性的人工生命现象量化,从而通过自动化这一传统上依赖人工且耗时的过程,加速了研究进展。作者通过实验验证了 ASAL 的有效性,并讨论了其未来应用,包括扩展到视频和 3D 模拟领域。论文还探讨了使用不同基础模型和基质的影响,强调为特定研究目标选择合适模型的重要性。
原文链接:https://arxiv.org/abs/2412.17799
Seventy3: 用NotebookLM将论文生成播客,让大家跟着AI一起进步。
今天的主题是:Automating the Search for Artificial Life with Foundation ModelsSummary
This research paper introduces Automated Search for Artificial Life (ASAL), a novel method using foundation models (FMs) to automate the discovery of interesting artificial life (ALife) simulations. ASAL employs FMs to evaluate simulations across diverse substrates (like Boids and Lenia), enabling three search strategies: supervised target searching, open-endedness searching, and illumination. The approach successfully discovers unseen lifeforms and quantifies previously qualitative ALife phenomena, accelerating research by automating a traditionally manual and time-consuming process. The authors demonstrate ASAL's effectiveness through experiments and discuss future applications, including expanding to video and 3D simulations. Finally, the paper explores the implications of using different FMs and substrates, highlighting the importance of selecting appropriate models for specific research goals.
本文提出了自动化人工生命搜索(Automated Search for Artificial Life,ASAL),一种利用基础模型(FMs)自动发现有趣人工生命(ALife)模拟的新方法。ASAL 利用基础模型在多样化基质(如 Boids 和 Lenia)上评估模拟,支持三种搜索策略:有监督目标搜索、开放性搜索和照明搜索。该方法成功发现了未曾见过的生命形式,并将以往定性的人工生命现象量化,从而通过自动化这一传统上依赖人工且耗时的过程,加速了研究进展。作者通过实验验证了 ASAL 的有效性,并讨论了其未来应用,包括扩展到视频和 3D 模拟领域。论文还探讨了使用不同基础模型和基质的影响,强调为特定研究目标选择合适模型的重要性。
原文链接:https://arxiv.org/abs/2412.17799