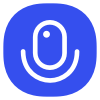
Sign up to save your podcasts
Or
Seventy3: 用NotebookLM将论文生成播客,让大家跟着AI一起进步。
今天的主题是:Explore Theory of Mind: Program-guided adversarial data generation for theory of mind reasoningSummary
The paper introduces ExploreToM, a novel framework for generating complex and diverse theory-of-mind (ToM) datasets for evaluating and training large language models (LLMs). ExploreToM uses an A* search algorithm and a domain-specific language to create challenging story scenarios, revealing significant weaknesses in current LLMs' ToM abilities. The generated data, available online, demonstrates that state-of-the-art LLMs struggle with fundamental skills like state tracking and show surprisingly low accuracy on the generated tasks. Fine-tuning LLMs on ExploreToM data significantly improves their performance on existing ToM benchmarks, highlighting the framework's utility for advancing ToM research. The authors also explore the underlying reasons for LLMs' poor ToM performance, pointing to data biases and the need for targeted training.
本文提出了ExploreToM,一种用于生成复杂且多样化的心智理论(Theory of Mind, ToM)数据集的新框架,以评估和训练大型语言模型(LLMs)。ExploreToM 利用 A* 搜索算法和领域特定语言创建具有挑战性的故事场景,揭示了当前 LLM 在 ToM 能力上的显著不足。生成的数据集已在线公开,表明最先进的 LLM 在诸如状态跟踪等基本技能上表现不佳,并且在这些任务上的准确率意外地低。将 LLM 在 ExploreToM 数据集上进行微调后,其在现有 ToM 基准测试中的表现显著提升,突显了该框架对推进 ToM 研究的价值。作者还探讨了 LLM 在 ToM 表现不佳的潜在原因,指出数据偏差以及对有针对性训练的需求。
原文链接:https://arxiv.org/abs/2412.12175
Seventy3: 用NotebookLM将论文生成播客,让大家跟着AI一起进步。
今天的主题是:Explore Theory of Mind: Program-guided adversarial data generation for theory of mind reasoningSummary
The paper introduces ExploreToM, a novel framework for generating complex and diverse theory-of-mind (ToM) datasets for evaluating and training large language models (LLMs). ExploreToM uses an A* search algorithm and a domain-specific language to create challenging story scenarios, revealing significant weaknesses in current LLMs' ToM abilities. The generated data, available online, demonstrates that state-of-the-art LLMs struggle with fundamental skills like state tracking and show surprisingly low accuracy on the generated tasks. Fine-tuning LLMs on ExploreToM data significantly improves their performance on existing ToM benchmarks, highlighting the framework's utility for advancing ToM research. The authors also explore the underlying reasons for LLMs' poor ToM performance, pointing to data biases and the need for targeted training.
本文提出了ExploreToM,一种用于生成复杂且多样化的心智理论(Theory of Mind, ToM)数据集的新框架,以评估和训练大型语言模型(LLMs)。ExploreToM 利用 A* 搜索算法和领域特定语言创建具有挑战性的故事场景,揭示了当前 LLM 在 ToM 能力上的显著不足。生成的数据集已在线公开,表明最先进的 LLM 在诸如状态跟踪等基本技能上表现不佳,并且在这些任务上的准确率意外地低。将 LLM 在 ExploreToM 数据集上进行微调后,其在现有 ToM 基准测试中的表现显著提升,突显了该框架对推进 ToM 研究的价值。作者还探讨了 LLM 在 ToM 表现不佳的潜在原因,指出数据偏差以及对有针对性训练的需求。
原文链接:https://arxiv.org/abs/2412.12175