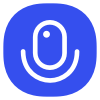
Sign up to save your podcasts
Or
Seventy3: 用NotebookLM将论文生成播客,让大家跟着AI一起进步。
今天的主题是:Free Process Rewards without Process LabelsSummary
This research paper proposes a cost-effective method for training process reward models (PRMs), which evaluate the intermediate steps of a reasoning process. Unlike existing PRMs requiring costly step-level labels, the authors demonstrate that a strong PRM can be implicitly learned at no extra cost by training an outcome reward model (ORM) with a specific reward parameterization. Their method, termed "implicit PRM," outperforms existing baselines on mathematical reasoning tasks while significantly reducing data collection and training overhead. Experiments explore various instantiations of the implicit PRM with different loss functions, showing consistent improvements and data efficiency. The findings suggest a paradigm shift in PRM training approaches, making them more accessible for broader applications.
这篇研究论文提出了一种具有成本效益的训练过程奖励模型(PRMs)的方法,该模型用于评估推理过程中的中间步骤。与现有需要高成本步骤级标签的 PRM 不同,作者展示了一种通过特定奖励参数化训练结果奖励模型(ORM)来隐式学习强大的 PRM,且没有额外成本。该方法被称为“隐式 PRM”,在数学推理任务中优于现有基准,并显著减少了数据收集和训练开销。实验探索了使用不同损失函数的隐式 PRM 的各种实现,显示出一致的性能提升和数据效率。这些发现表明,PRM 训练方法可能迎来范式转变,使其在更广泛的应用中变得更加可访问。
原文链接:https://arxiv.org/abs/2412.01981
Seventy3: 用NotebookLM将论文生成播客,让大家跟着AI一起进步。
今天的主题是:Free Process Rewards without Process LabelsSummary
This research paper proposes a cost-effective method for training process reward models (PRMs), which evaluate the intermediate steps of a reasoning process. Unlike existing PRMs requiring costly step-level labels, the authors demonstrate that a strong PRM can be implicitly learned at no extra cost by training an outcome reward model (ORM) with a specific reward parameterization. Their method, termed "implicit PRM," outperforms existing baselines on mathematical reasoning tasks while significantly reducing data collection and training overhead. Experiments explore various instantiations of the implicit PRM with different loss functions, showing consistent improvements and data efficiency. The findings suggest a paradigm shift in PRM training approaches, making them more accessible for broader applications.
这篇研究论文提出了一种具有成本效益的训练过程奖励模型(PRMs)的方法,该模型用于评估推理过程中的中间步骤。与现有需要高成本步骤级标签的 PRM 不同,作者展示了一种通过特定奖励参数化训练结果奖励模型(ORM)来隐式学习强大的 PRM,且没有额外成本。该方法被称为“隐式 PRM”,在数学推理任务中优于现有基准,并显著减少了数据收集和训练开销。实验探索了使用不同损失函数的隐式 PRM 的各种实现,显示出一致的性能提升和数据效率。这些发现表明,PRM 训练方法可能迎来范式转变,使其在更广泛的应用中变得更加可访问。
原文链接:https://arxiv.org/abs/2412.01981