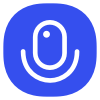
Sign up to save your podcasts
Or
Seventy3: 用NotebookLM将论文生成播客,让大家跟着AI一起进步。
今天的主题是:Metadata Conditioning Accelerates Language Model Pre-trainingSummary
This research paper introduces Metadata Conditioning then Cooldown (MeCo), a novel method for improving the efficiency and controllability of large language model pre-training. MeCo incorporates readily available metadata, such as URLs, to enhance the model's understanding of diverse data sources during training, then uses a "cooldown" phase to ensure functionality without metadata during inference. Experiments demonstrate that MeCo significantly accelerates pre-training, achieving comparable performance with less data and enabling better control over model outputs by conditioning inference prompts with metadata. The study explores various metadata types and ablates design choices to understand MeCo's effectiveness, showcasing its potential for creating more capable and steerable language models. Finally, the paper compares MeCo to existing techniques for data selection and metadata conditioning.
这篇研究论文介绍了一种新颖的方法——元数据调节与冷却(Metadata Conditioning then Cooldown,简称 MeCo),旨在提高大规模语言模型预训练的效率和可控性。MeCo 利用现成的元数据(如 URL)来增强模型在训练过程中对多样化数据源的理解,然后通过“冷却”阶段确保推理时不依赖元数据。实验表明,MeCo 能显著加速预训练,在使用更少数据的情况下实现相当的性能,并通过将推理提示与元数据结合来更好地控制模型输出。研究还探索了各种元数据类型,并通过消融实验分析了设计选择,以理解 MeCo 的有效性,展示了它在创建更强大且可调控语言模型方面的潜力。最后,论文将 MeCo 与现有的数据选择和元数据调节技术进行了比较。
原文链接:https://arxiv.org/abs/2501.01956
Seventy3: 用NotebookLM将论文生成播客,让大家跟着AI一起进步。
今天的主题是:Metadata Conditioning Accelerates Language Model Pre-trainingSummary
This research paper introduces Metadata Conditioning then Cooldown (MeCo), a novel method for improving the efficiency and controllability of large language model pre-training. MeCo incorporates readily available metadata, such as URLs, to enhance the model's understanding of diverse data sources during training, then uses a "cooldown" phase to ensure functionality without metadata during inference. Experiments demonstrate that MeCo significantly accelerates pre-training, achieving comparable performance with less data and enabling better control over model outputs by conditioning inference prompts with metadata. The study explores various metadata types and ablates design choices to understand MeCo's effectiveness, showcasing its potential for creating more capable and steerable language models. Finally, the paper compares MeCo to existing techniques for data selection and metadata conditioning.
这篇研究论文介绍了一种新颖的方法——元数据调节与冷却(Metadata Conditioning then Cooldown,简称 MeCo),旨在提高大规模语言模型预训练的效率和可控性。MeCo 利用现成的元数据(如 URL)来增强模型在训练过程中对多样化数据源的理解,然后通过“冷却”阶段确保推理时不依赖元数据。实验表明,MeCo 能显著加速预训练,在使用更少数据的情况下实现相当的性能,并通过将推理提示与元数据结合来更好地控制模型输出。研究还探索了各种元数据类型,并通过消融实验分析了设计选择,以理解 MeCo 的有效性,展示了它在创建更强大且可调控语言模型方面的潜力。最后,论文将 MeCo 与现有的数据选择和元数据调节技术进行了比较。
原文链接:https://arxiv.org/abs/2501.01956