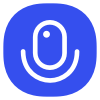
Sign up to save your podcasts
Or
Seventy3: 用NotebookLM将论文生成播客,让大家跟着AI一起进步。
今天的主题是:OS-Genesis: Automating GUI Agent Trajectory Construction via Reverse Task SynthesisSummary
This research paper introduces OS-Genesis, a novel pipeline for synthesizing high-quality and diverse data for training Graphical User Interface (GUI) agents. Unlike existing methods that rely on pre-defined tasks or human supervision, OS-Genesis uses an interaction-driven approach, allowing agents to explore environments and retrospectively derive tasks. A trajectory reward model ensures data quality, and experiments demonstrate OS-Genesis's superior performance on challenging benchmarks. The authors also analyze data diversity and the impact of the reward model. Finally, they discuss OS-Genesis' limitations and broader implications for digital automation.
这篇研究论文介绍了OS-Genesis,一种新颖的数据合成流程,用于训练图形用户界面(GUI)代理。与依赖于预定义任务或人工监督的现有方法不同,OS-Genesis 采用互动驱动的方法,允许代理在环境中进行探索,并从中回溯推导任务。轨迹奖励模型确保数据质量,实验表明 OS-Genesis 在具有挑战性的基准测试中表现优异。作者还分析了数据多样性和奖励模型的影响。最后,论文讨论了 OS-Genesis 的局限性及其在数字自动化领域的更广泛意义。
原文链接:https://arxiv.org/abs/2412.19723
Seventy3: 用NotebookLM将论文生成播客,让大家跟着AI一起进步。
今天的主题是:OS-Genesis: Automating GUI Agent Trajectory Construction via Reverse Task SynthesisSummary
This research paper introduces OS-Genesis, a novel pipeline for synthesizing high-quality and diverse data for training Graphical User Interface (GUI) agents. Unlike existing methods that rely on pre-defined tasks or human supervision, OS-Genesis uses an interaction-driven approach, allowing agents to explore environments and retrospectively derive tasks. A trajectory reward model ensures data quality, and experiments demonstrate OS-Genesis's superior performance on challenging benchmarks. The authors also analyze data diversity and the impact of the reward model. Finally, they discuss OS-Genesis' limitations and broader implications for digital automation.
这篇研究论文介绍了OS-Genesis,一种新颖的数据合成流程,用于训练图形用户界面(GUI)代理。与依赖于预定义任务或人工监督的现有方法不同,OS-Genesis 采用互动驱动的方法,允许代理在环境中进行探索,并从中回溯推导任务。轨迹奖励模型确保数据质量,实验表明 OS-Genesis 在具有挑战性的基准测试中表现优异。作者还分析了数据多样性和奖励模型的影响。最后,论文讨论了 OS-Genesis 的局限性及其在数字自动化领域的更广泛意义。
原文链接:https://arxiv.org/abs/2412.19723