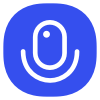
Sign up to save your podcasts
Or
Seventy3: 用NotebookLM将论文生成播客,让大家跟着AI一起进步。
今天的主题是:Towards System 2 Reasoning in LLMs: Learning How to Think With Meta Chain-of-ThoughtSummary
The paper "Towards System 2 Reasoning in LLMs" explores methods for improving the reasoning capabilities of large language models (LLMs). It introduces Meta Chain-of-Thought (Meta-CoT), a framework that models the reasoning process itself, going beyond traditional Chain-of-Thought prompting. The authors investigate using search algorithms, synthetic data, and reinforcement learning to train models that generate Meta-CoTs. Empirical results and scaling laws related to inference-time computation and the generator-verifier gap are presented, along with open research questions regarding the emergence of more human-like reasoning in AI. The included example problem-solving attempts illustrate different approaches to this challenge.
论文《朝着系统2推理的方向发展》探讨了提升大语言模型(LLMs)推理能力的方法。文章提出了“元思维链”(Meta Chain-of-Thought,Meta-CoT)框架,该框架将推理过程本身建模,超越了传统的思维链提示方法。作者研究了使用搜索算法、合成数据和强化学习来训练生成Meta-CoT的模型。文章展示了与推理时计算和生成器-验证器差距相关的经验结果和扩展法则,并提出了关于AI中更类似人类推理出现的开放研究问题。文中所包含的示例问题解决尝试展示了应对这一挑战的不同方法。
原文链接:https://arxiv.org/abs/2501.04682
Seventy3: 用NotebookLM将论文生成播客,让大家跟着AI一起进步。
今天的主题是:Towards System 2 Reasoning in LLMs: Learning How to Think With Meta Chain-of-ThoughtSummary
The paper "Towards System 2 Reasoning in LLMs" explores methods for improving the reasoning capabilities of large language models (LLMs). It introduces Meta Chain-of-Thought (Meta-CoT), a framework that models the reasoning process itself, going beyond traditional Chain-of-Thought prompting. The authors investigate using search algorithms, synthetic data, and reinforcement learning to train models that generate Meta-CoTs. Empirical results and scaling laws related to inference-time computation and the generator-verifier gap are presented, along with open research questions regarding the emergence of more human-like reasoning in AI. The included example problem-solving attempts illustrate different approaches to this challenge.
论文《朝着系统2推理的方向发展》探讨了提升大语言模型(LLMs)推理能力的方法。文章提出了“元思维链”(Meta Chain-of-Thought,Meta-CoT)框架,该框架将推理过程本身建模,超越了传统的思维链提示方法。作者研究了使用搜索算法、合成数据和强化学习来训练生成Meta-CoT的模型。文章展示了与推理时计算和生成器-验证器差距相关的经验结果和扩展法则,并提出了关于AI中更类似人类推理出现的开放研究问题。文中所包含的示例问题解决尝试展示了应对这一挑战的不同方法。
原文链接:https://arxiv.org/abs/2501.04682