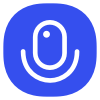
Sign up to save your podcasts
Or
Seventy3: 用NotebookLM将论文生成播客,让大家跟着AI一起进步。
今天的主题是:DPO Kernels: A Semantically-Aware, Kernel-Enhanced, and Divergence-Rich Paradigm for Direct Preference OptimizationSummary
This research paper introduces DPO-Kernels, an improved method for aligning large language models (LLMs) with human preferences. It enhances Direct Preference Optimization (DPO) by incorporating kernel methods for richer feature transformations and diverse divergence measures for increased robustness. A data-driven approach automatically selects the optimal kernel-divergence pair, eliminating manual tuning. Furthermore, a Hierarchical Mixture of Kernels (HMK) framework combines local and global kernels to balance fine-grained and large-scale dependencies. The paper also explores generalization, overfitting, and ethical considerations related to fairness, bias, and privacy.
这篇研究论文介绍了DPO-Kernels,一种改进的大型语言模型(LLMs)与人类偏好对齐的方法。它通过结合核方法来增强直接偏好优化(DPO),实现了更丰富的特征转换,并采用多样的散度度量来提高模型的鲁棒性。该方法采用数据驱动的方式自动选择最优的核-散度对,从而避免了手动调整。此外,论文提出了一种层次化的核混合(HMK)框架,结合了局部和全局核,以平衡细粒度和大规模依赖关系。论文还探讨了泛化能力、过拟合问题以及与公平性、偏见和隐私相关的伦理考虑。
原文链接:https://arxiv.org/abs/2501.03271
Seventy3: 用NotebookLM将论文生成播客,让大家跟着AI一起进步。
今天的主题是:DPO Kernels: A Semantically-Aware, Kernel-Enhanced, and Divergence-Rich Paradigm for Direct Preference OptimizationSummary
This research paper introduces DPO-Kernels, an improved method for aligning large language models (LLMs) with human preferences. It enhances Direct Preference Optimization (DPO) by incorporating kernel methods for richer feature transformations and diverse divergence measures for increased robustness. A data-driven approach automatically selects the optimal kernel-divergence pair, eliminating manual tuning. Furthermore, a Hierarchical Mixture of Kernels (HMK) framework combines local and global kernels to balance fine-grained and large-scale dependencies. The paper also explores generalization, overfitting, and ethical considerations related to fairness, bias, and privacy.
这篇研究论文介绍了DPO-Kernels,一种改进的大型语言模型(LLMs)与人类偏好对齐的方法。它通过结合核方法来增强直接偏好优化(DPO),实现了更丰富的特征转换,并采用多样的散度度量来提高模型的鲁棒性。该方法采用数据驱动的方式自动选择最优的核-散度对,从而避免了手动调整。此外,论文提出了一种层次化的核混合(HMK)框架,结合了局部和全局核,以平衡细粒度和大规模依赖关系。论文还探讨了泛化能力、过拟合问题以及与公平性、偏见和隐私相关的伦理考虑。
原文链接:https://arxiv.org/abs/2501.03271