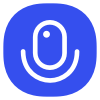
Sign up to save your podcasts
Or
Seventy3: 用NotebookLM将论文生成播客,让大家跟着AI一起进步。
今天的主题是:The GAN is dead; long live the GAN! A Modern GAN BaselineSummary
This NeurIPS 2024 paper introduces R3GAN, a simplified Generative Adversarial Network (GAN) that achieves state-of-the-art performance. The authors achieve this by developing a novel, mathematically well-behaved loss function that eliminates the need for the ad-hoc training tricks common in previous GANs. This improved loss enables the use of modern neural network architectures, resulting in a more efficient and effective model. R3GAN surpasses existing GANs and diffusion models on several benchmark datasets, demonstrating the effectiveness of the proposed approach. The paper rigorously supports its claims through mathematical analysis and extensive empirical results. The authors also discuss the limitations of their approach and potential societal impacts of GAN technology.
这篇NeurIPS 2024论文介绍了R3GAN,一种简化的生成对抗网络(GAN),实现了当前的最先进性能。作者通过开发一种新颖的、数学上表现良好的损失函数,消除了以往GAN中常见的临时训练技巧。这种改进的损失函数使得能够使用现代神经网络架构,从而使得模型更加高效和有效。R3GAN在多个基准数据集上超越了现有的GAN和扩散模型,展示了该方法的有效性。论文通过数学分析和大量实证结果严密支持其论点。作者还讨论了该方法的局限性以及GAN技术可能对社会带来的影响。
原文链接:https://arxiv.org/abs/2501.05441
Seventy3: 用NotebookLM将论文生成播客,让大家跟着AI一起进步。
今天的主题是:The GAN is dead; long live the GAN! A Modern GAN BaselineSummary
This NeurIPS 2024 paper introduces R3GAN, a simplified Generative Adversarial Network (GAN) that achieves state-of-the-art performance. The authors achieve this by developing a novel, mathematically well-behaved loss function that eliminates the need for the ad-hoc training tricks common in previous GANs. This improved loss enables the use of modern neural network architectures, resulting in a more efficient and effective model. R3GAN surpasses existing GANs and diffusion models on several benchmark datasets, demonstrating the effectiveness of the proposed approach. The paper rigorously supports its claims through mathematical analysis and extensive empirical results. The authors also discuss the limitations of their approach and potential societal impacts of GAN technology.
这篇NeurIPS 2024论文介绍了R3GAN,一种简化的生成对抗网络(GAN),实现了当前的最先进性能。作者通过开发一种新颖的、数学上表现良好的损失函数,消除了以往GAN中常见的临时训练技巧。这种改进的损失函数使得能够使用现代神经网络架构,从而使得模型更加高效和有效。R3GAN在多个基准数据集上超越了现有的GAN和扩散模型,展示了该方法的有效性。论文通过数学分析和大量实证结果严密支持其论点。作者还讨论了该方法的局限性以及GAN技术可能对社会带来的影响。
原文链接:https://arxiv.org/abs/2501.05441