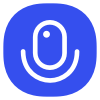
Sign up to save your podcasts
Or
Seventy3: 用NotebookLM将论文生成播客,让大家跟着AI一起进步。
今天的主题是:CLIP-guided continual novel class discoverySummary
This research paper introduces a novel method for Continual Novel Class Discovery (CNCD), a challenging machine learning problem focusing on teaching a model new classes without forgetting previously learned ones, especially when old data is unavailable. The proposed method leverages the CLIP model for guidance in identifying new classes and uses techniques like CutMix and prototype adaptation to improve representation learning and prevent forgetting. Experiments on several benchmark datasets demonstrate the method's effectiveness in balancing the learning of both new and old classes. The paper also explores the benefits of decoupling the training process for old and new classes and compares its performance to existing CNCD and novel class discovery methods. The authors conclude by discussing limitations and future directions for improving computational efficiency.
这篇研究论文介绍了一种新颖的持续新类发现(CNCD)方法,这是一种具有挑战性的机器学习问题,主要集中在如何在没有旧数据的情况下,教授模型识别新类别而不忘记已学过的类别。所提方法利用CLIP模型为识别新类别提供指导,并采用诸如CutMix和原型适应等技术来提升表示学习和防止遗忘。在多个基准数据集上的实验表明,该方法在平衡新旧类别学习方面具有良好的效果。论文还探讨了将旧类别和新类别的训练过程解耦的好处,并将其与现有的CNCD和新类发现方法进行了比较。作者最后讨论了方法的局限性以及在提升计算效率方面的未来发展方向。
原文链接:https://www.sciencedirect.com/science/article/abs/pii/S0950705124015545
Seventy3: 用NotebookLM将论文生成播客,让大家跟着AI一起进步。
今天的主题是:CLIP-guided continual novel class discoverySummary
This research paper introduces a novel method for Continual Novel Class Discovery (CNCD), a challenging machine learning problem focusing on teaching a model new classes without forgetting previously learned ones, especially when old data is unavailable. The proposed method leverages the CLIP model for guidance in identifying new classes and uses techniques like CutMix and prototype adaptation to improve representation learning and prevent forgetting. Experiments on several benchmark datasets demonstrate the method's effectiveness in balancing the learning of both new and old classes. The paper also explores the benefits of decoupling the training process for old and new classes and compares its performance to existing CNCD and novel class discovery methods. The authors conclude by discussing limitations and future directions for improving computational efficiency.
这篇研究论文介绍了一种新颖的持续新类发现(CNCD)方法,这是一种具有挑战性的机器学习问题,主要集中在如何在没有旧数据的情况下,教授模型识别新类别而不忘记已学过的类别。所提方法利用CLIP模型为识别新类别提供指导,并采用诸如CutMix和原型适应等技术来提升表示学习和防止遗忘。在多个基准数据集上的实验表明,该方法在平衡新旧类别学习方面具有良好的效果。论文还探讨了将旧类别和新类别的训练过程解耦的好处,并将其与现有的CNCD和新类发现方法进行了比较。作者最后讨论了方法的局限性以及在提升计算效率方面的未来发展方向。
原文链接:https://www.sciencedirect.com/science/article/abs/pii/S0950705124015545