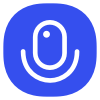
Sign up to save your podcasts
Or
Seventy3: 用NotebookLM将论文生成播客,让大家跟着AI一起进步。
今天的主题是:Titans: Learning to Memorize at Test TimeSummary
This research paper introduces Titans, a novel family of neural architectures designed to improve long-term memory in sequence modeling. Titans incorporate a new neural long-term memory module that learns to memorize historical context at test time, addressing the limitations of Transformers and existing recurrent models. The model uses a "surprise" metric to determine what information to remember and a forgetting mechanism to manage memory capacity. Three Titans variants—Memory as a Context, Memory as a Gate, and Memory as a Layer—are presented, showcasing different ways to integrate the long-term memory module. Experimental results across various tasks demonstrate Titans' superior performance and scalability to extremely long contexts.
这篇研究论文介绍了Titans,一种新型神经网络架构家族,旨在改善序列建模中的长期记忆。Titans引入了一个新的神经长期记忆模块,能够在测试时学习记住历史上下文,解决了Transformer和现有循环模型的局限性。该模型使用“惊讶”度量来决定记住哪些信息,并采用遗忘机制来管理记忆容量。论文提出了三种Titans变体——“记忆作为上下文”、“记忆作为门控”和“记忆作为层”,展示了集成长期记忆模块的不同方式。跨多个任务的实验结果表明,Titans在处理极长上下文时表现出色,并具有更强的扩展性。
原文链接:https://arxiv.org/abs/2501.00663
Seventy3: 用NotebookLM将论文生成播客,让大家跟着AI一起进步。
今天的主题是:Titans: Learning to Memorize at Test TimeSummary
This research paper introduces Titans, a novel family of neural architectures designed to improve long-term memory in sequence modeling. Titans incorporate a new neural long-term memory module that learns to memorize historical context at test time, addressing the limitations of Transformers and existing recurrent models. The model uses a "surprise" metric to determine what information to remember and a forgetting mechanism to manage memory capacity. Three Titans variants—Memory as a Context, Memory as a Gate, and Memory as a Layer—are presented, showcasing different ways to integrate the long-term memory module. Experimental results across various tasks demonstrate Titans' superior performance and scalability to extremely long contexts.
这篇研究论文介绍了Titans,一种新型神经网络架构家族,旨在改善序列建模中的长期记忆。Titans引入了一个新的神经长期记忆模块,能够在测试时学习记住历史上下文,解决了Transformer和现有循环模型的局限性。该模型使用“惊讶”度量来决定记住哪些信息,并采用遗忘机制来管理记忆容量。论文提出了三种Titans变体——“记忆作为上下文”、“记忆作为门控”和“记忆作为层”,展示了集成长期记忆模块的不同方式。跨多个任务的实验结果表明,Titans在处理极长上下文时表现出色,并具有更强的扩展性。
原文链接:https://arxiv.org/abs/2501.00663